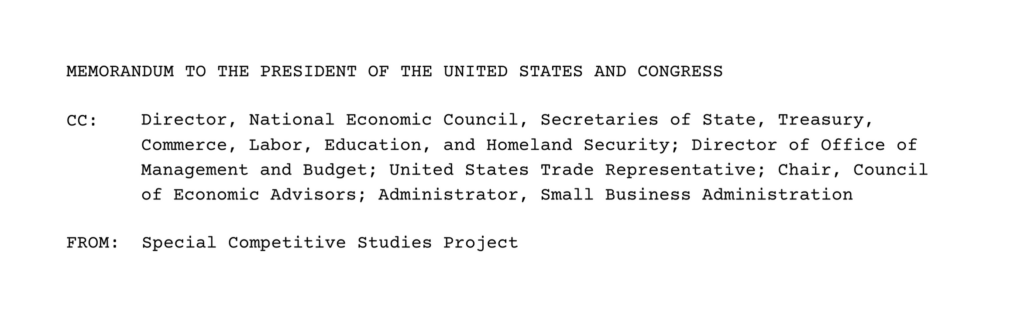
Executive Summary
While the United States is currently the leader in generative artificial intelligence (GenAI), there is no guarantee that we will remain in the lead without a focused national effort. Our primary rival for AI leadership, the People’s Republic of China (PRC), understands the strategic and economic significance of AI and has a track record of executing well-resourced technology strategies that have produced results.1 Nations that harness the potential of GenAI will increase productivity, a key economic measure that underpins economic growth, higher standards of living, and the ability to finance all other national priorities. To unlock these benefits, the United States should bolster competitiveness across the fundamental building blocks that underpin AI leadership.
- Compute: The United States should assure access to compute resources via cloud providers, encourage sustainable AI techniques, protect U.S. advantages in microelectronics, and lead in accelerating hardware innovation.
- Data: The United States should develop a whole-of-nation strategy for digital infrastructure and create a trusted regulatory framework that harnesses data for social and economic benefit while paving the way to increased digital trade with allies and partners.
- People: The United States should pursue immigration reforms to continue to attract top AI talent, establish a National Commission on Automation and the Future of Work, and develop curriculum guidelines to safely deploy GenAI in the classroom.
This memo outlines 1) the economic implications of GenAI and 2) steps the United States can take, along with allies and partners, to capture its full potential and sustain democratic leadership in this future-shaping technology.
Introduction
GenAI is poised to transform the global economy. Some paint a dystopian future in which deploying generative systems causes mass layoffs, rising inequality, and social instability. Others believe that this technology heralds the dawn of a fourth industrial revolution that will drive tremendous economic gains, enhancing human welfare while reversing years of deceleration in productivity growth in the United States and other advanced economies.2
The introduction of generative AI also comes at a time when the global economy is already undergoing transformative changes. Nations around the world are investing heavily in technology competitiveness while pursuing “de-risking” policies meant to build resilience for critical technology inputs. Companies, similarly, are pursuing diversification to reduce overreliance on China. While trade and technology guardrails in strategic sectors have become commonplace, digital connectivity between nations is also accelerating.3 Depending on whether the impact of GenAI remains largely confined to service industries or extends deeply into manufacturing, this technology’s emergence could disrupt some of these trends, or it could accelerate them. It is simply too early to tell.
Ultimately, the future of the American economy – and, by extension, America’s international leadership and the vitality of democratic market economies around the world – could hinge on promoting American economic dynamism in this rapidly evolving sector. Economic growth is the prerequisite for pursuing all other national goals: reducing deficits, projecting military power, funding R&D and social programs, and strengthening ties with allies and partners.4 A GenAI-fueled productivity boom could bring more of these goals within reach.
Economic Implications
Increasing Productivity, Economic Output, and Welfare. Similar to previous general purpose technologies – such as the steam engine – GenAI could sharply increase the rate of technological change, paving the way for follow-on innovations that spark productivity improvements across every sector of the economy.5 However, unlike industrial technologies such as the steam engine, GenAI tools can perform a diverse range of functions and, given the intangible nature and relatively low cost of consumer software, face fewer barriers to adoption and diffusion.6 Analysts estimate that GenAI could deliver $4.4 trillion annually to the global economy — roughly equivalent to the GDP of Germany, the world’s fourth largest economy.7 Although these and other projections are not an exact science, they offer a sense of the potential magnitude of the impact of GenAI.8
Numerous corporations, including Oracle, Cisco, AT&T, Morgan Stanley, Crowdstrike, Amazon, Thomson Reuters, and Salesforce, are already leveraging GenAI to improve their products.9 Economists found that customer service agents at a Fortune 500 software firm with access to GenAI tools were 14 percent more productive on average than their unassisted colleagues.10 Evidence from experiments run by researchers at MIT suggest that generative AI “substantially raises” the average productivity of mid-level professionals with writing-intensive jobs.11 Meanwhile, GenAI is leading to breakthroughs in scientific research that would “otherwise take decades,” promising significant downstream economic significance.12
GenAI and the Federal Government. GenAI could improve organizational performance across the federal government, enabling departments and agencies to enhance the speed and quality of public services.13 The U.S. Patent and Trademark Office, for example, is already using new AI tools to enhance and accelerate the patent approval process and improve the accuracy of the trademark register.14
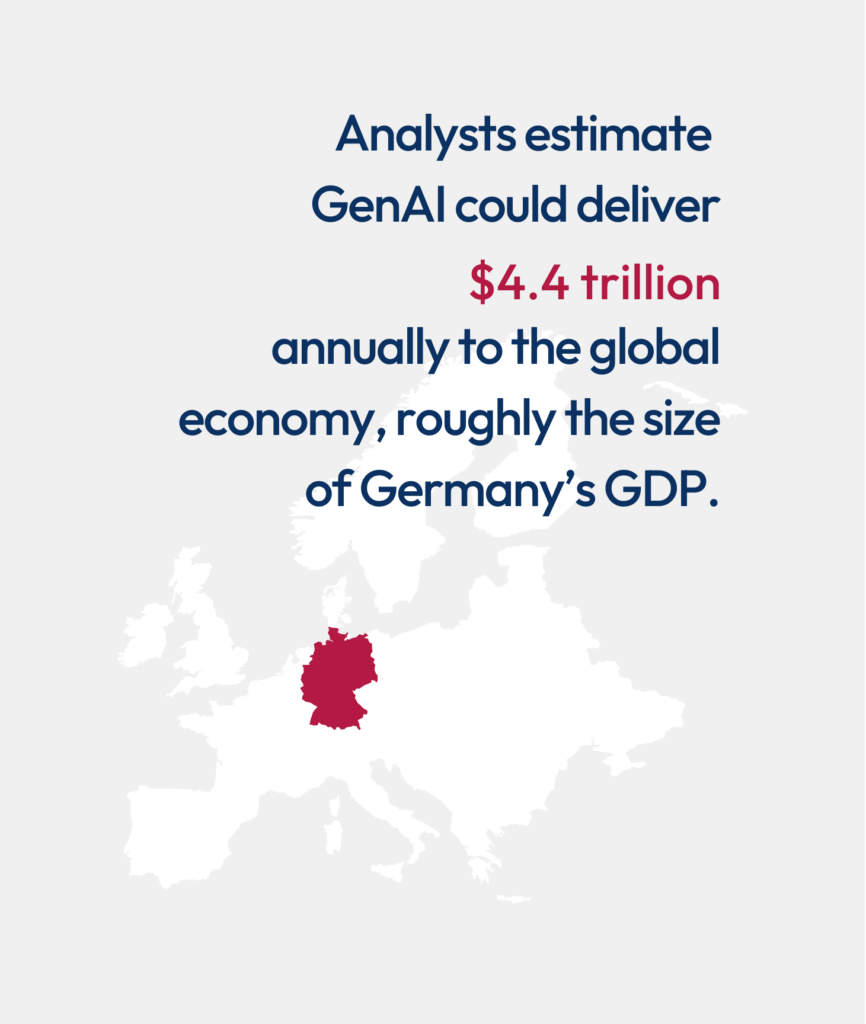
Potential Use Cases
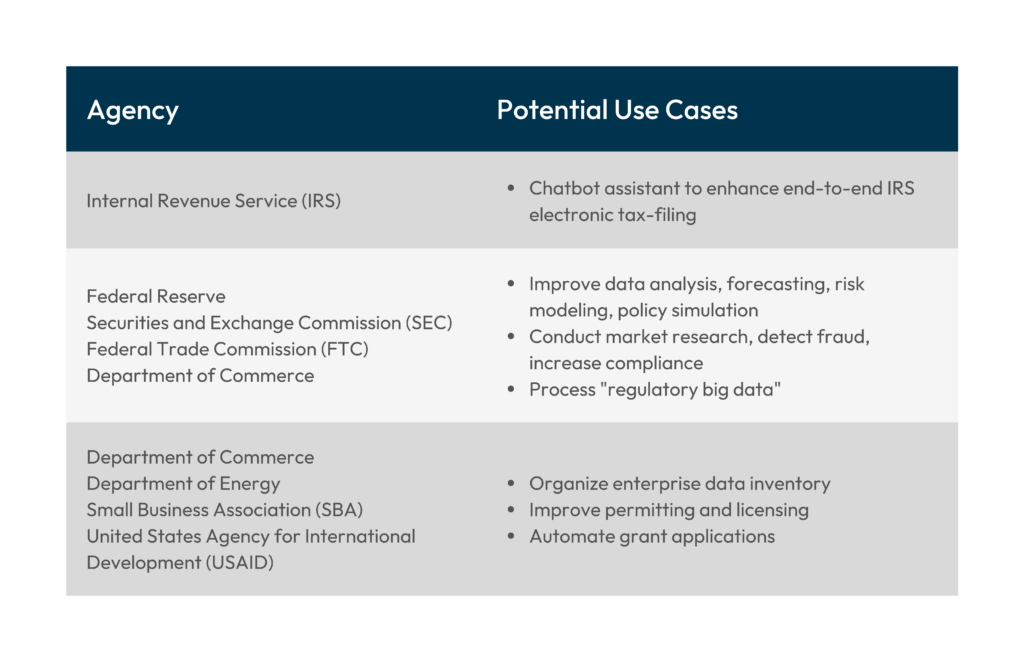
Impact on the Workforce. Exactly how and to what extent GenAI will impact the labor force remains an open question.15 Historical evidence suggests that automation technologies have, over the long run, created more jobs than they have displaced, and that they have made workers more productive across industries and professions, boosting productivity and per-capita GDP.16 Concerns have been raised, however, that this time could be different: workers could “lose economic and political bargaining power” at an unprecedented scale as AI threatens to eclipse not only human labor, but perhaps even human intelligence.17 Despite these anxieties, economists suggest that many professions, including low-wage occupations, are more likely to be “complemented rather than substituted by AI.”18 Scholars expect knowledge workers, in particular, to experience “groundbreaking shifts in their productivity within a few years” as a result of pervasive “cognitive automation.”19 More broadly, GenAI could address labor shortages that exist across various sectors of the economy.20
At this early stage, the economic consequences of recent advances in generative AI are difficult to project with certainty. However, one thing remains clear: maintaining democratic advantage vis-a-vis the PRC in this frontier technology requires a focused effort led by the United States and in collaboration with allies and partners.
Way Forward
GenAI leadership is built on three fundamental building blocks: compute, data, and people.21 Compute, or access to clusters of advanced AI chips, is the engine that powers GenAI. Sustaining innovation requires both large and small players to have access to compute resources. Data provides the raw material for training AI models and is a critical U.S. asset that must be harnessed in a way that protects Americans’ rights, including privacy, and complies with relevant legal regimes. Digital infrastructure like networking equipment and data centers is also vital for collecting, processing, and moving data. Finally, People – skilled AI researchers and end-users – are essential to advancing innovation, creating workforce opportunities, and sustaining national competitiveness.
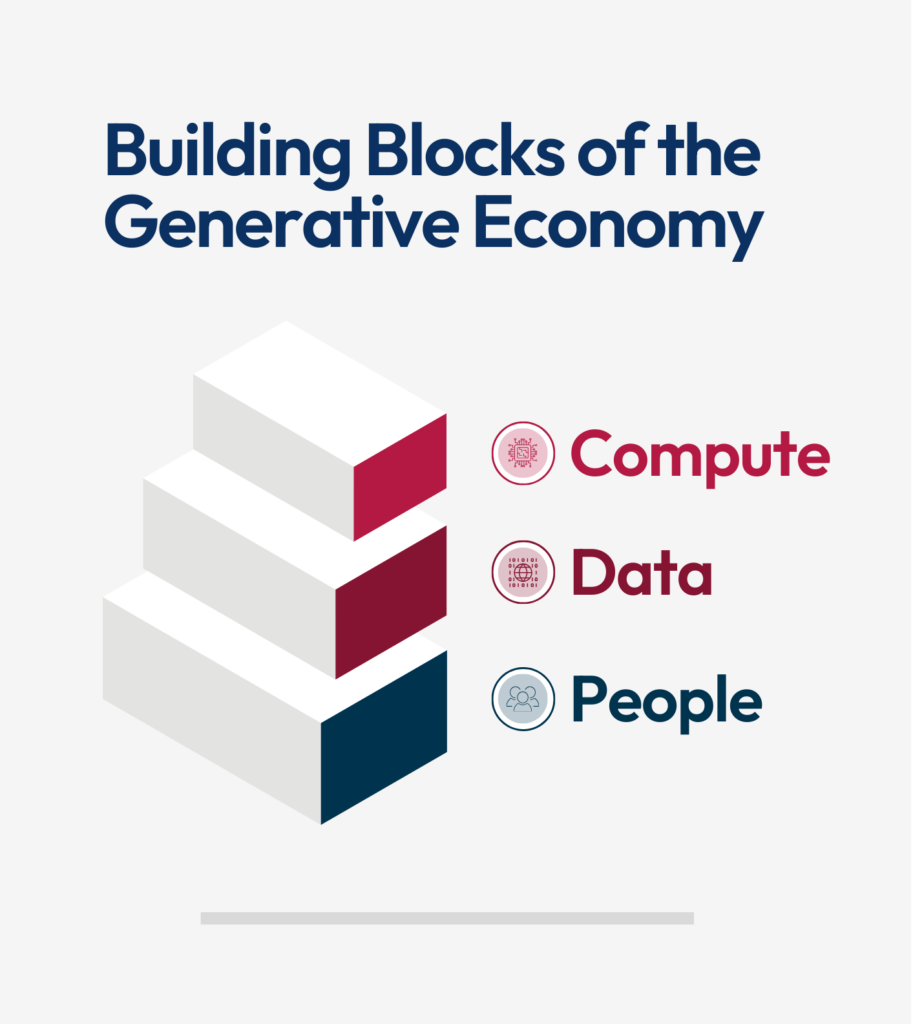
Compute
AI models require huge amounts of computing power, both for training runs and for inference (retrieval of information). Over the past decade, compute demand for AI has grown at an alarming rate. Since the first deep neural network-based AI model was demonstrated in 2012, the amount of compute resources needed to train frontier models has increased by roughly 55 million times – a doubling rate of about every six months.22 Today, the largest training runs take months and require supercomputers outfitted with thousands of specialized GPUs.23
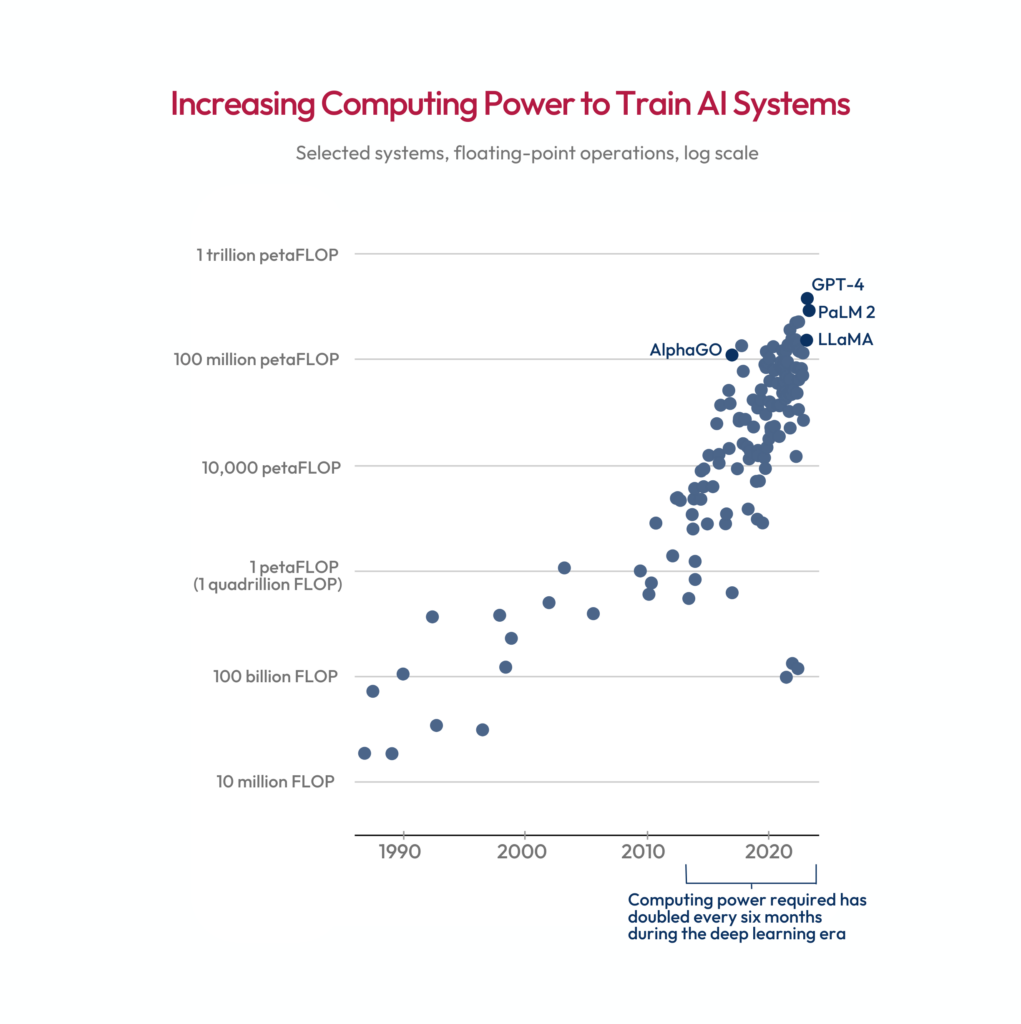
The United States enjoys a significant lead over the PRC in terms of both technological development and access to compute resources. Nvidia, the world’s leading designer of AI chips, is based in the United States, as are AMD and Intel, its two largest competitors.25 Last year, the United States announced restrictions on the export of cutting-edge AI chips and semiconductor manufacturing equipment to the PRC.26 Controls on the former apply only to the highest-end chips that meet thresholds for performance and interconnect speed. As a result, U.S. firms enjoy easier access to compute resources than PRC counterparts. However, well over 90 percent of the world’s AI chips are manufactured in Taiwan, leaving supply vulnerable for both countries.27 And while China is making compute resources available to academic researchers, the United States has not taken comparable measures.28
Compute Access & Cloud Computing
As noted above, when actors seek to train or deploy large models, access to computing power is a central consideration. Outside of large tech companies, most players – especially startups – access compute via cloud service providers.29 U.S. companies currently enjoy a substantial edge in cloud computing,30 although PRC cloud providers are actively attempting to undercut U.S. firms on price and are gaining market share in Asia, Latin America, and Africa.31 This U.S. lead is worth preserving. America’s leadership in cloud services provides a unique form of advantage that can be leveraged both in support of national competitiveness and to prevent bad actors from deploying dangerous models.32
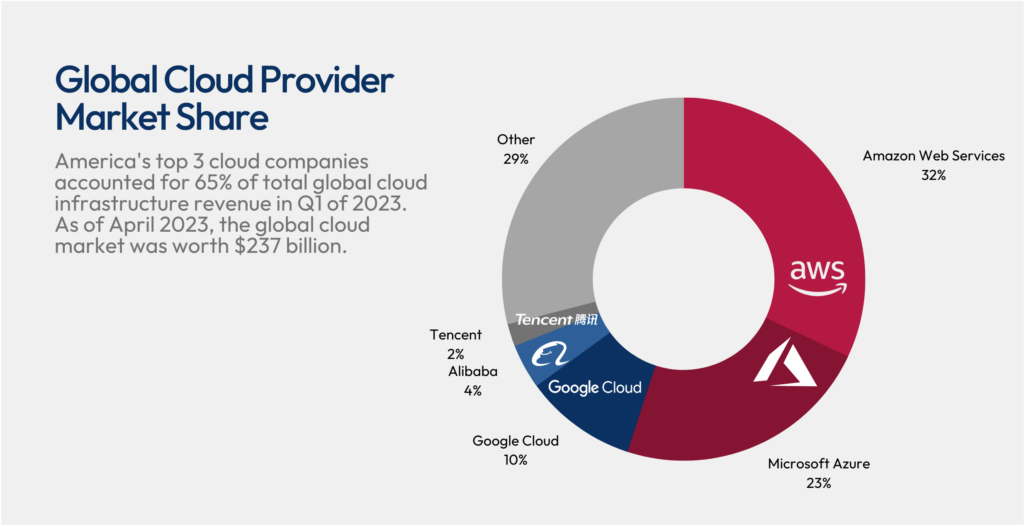
Source: Felix Richter, Big Three Dominate the Global Cloud Market, Statista (2023).
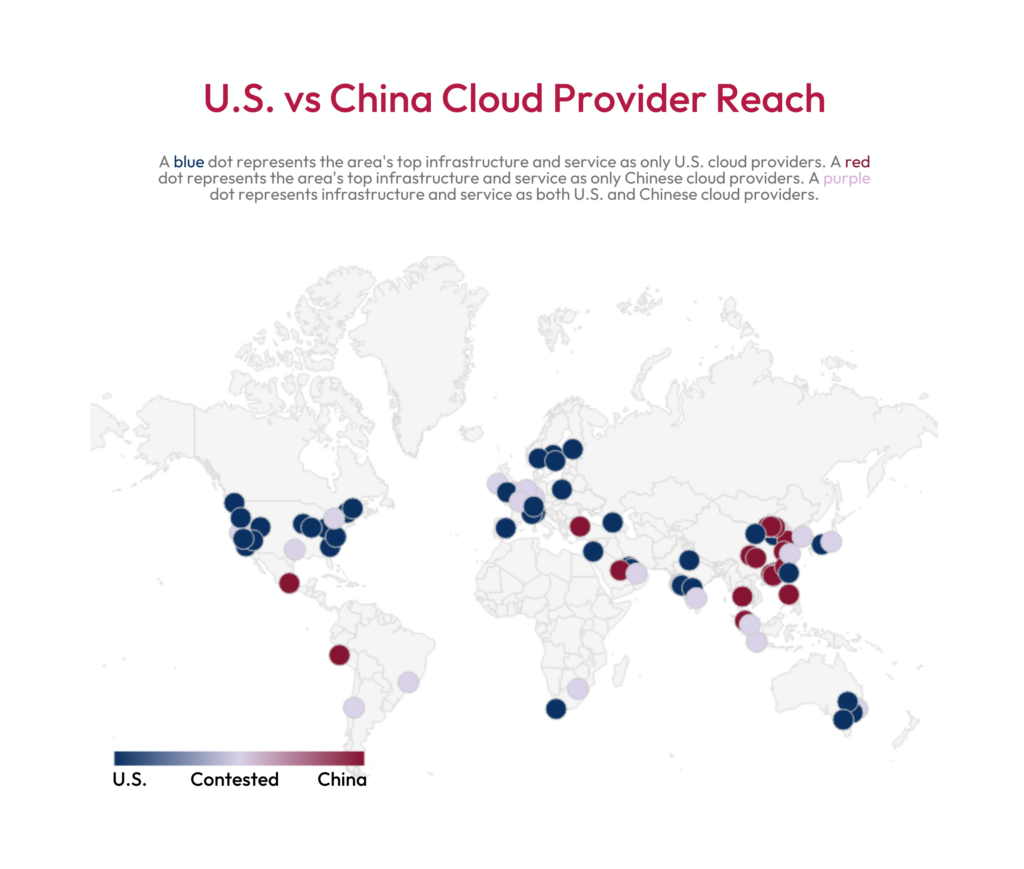
Key Actions
Create the National Artificial Intelligence Research Resource (NAIRR). To preserve America’s competitive edge in AI research and prevent expertise from becoming concentrated in a handful of companies, the United States must ensure academic researchers can access large amounts of compute resources via the cloud.34 The National Science Foundation (NSF) and OSTP, through their NAIRR Task Force, have released a detailed implementation plan for the NAIRR, supported by input from leading experts, academic institutions, and industry,35 and bipartisan, bicameral Members of Congress have introduced legislation to authorize the NAIRR.36 If authorized, this program should be funded as quickly as possible at $2.6 billion over six years, as requested by NSF and OSTP.37 The United States should also explore the creation of a parallel Democracies’ Artificial Intelligence Research Resource (DAIRR) in concert with key allies and partners.
Implement a know-your-customer regime for cloud providers. Recent reports suggest that sanctioned PRC AI companies are seeking to circumvent the October 7, 2022 restrictions by renting access to controlled GPUs via cloud providers.38 The Department of Commerce should begin by implementing know-your-customer requirements for U.S. cloud providers – as required by Executive Order 1398439 – as well as conducting diplomatic outreach with the Department of State to encourage allies and partners to implement responsible screening practices among their providers.40 These steps can be undertaken with existing resources and should be deployed as quickly as possible.
Potential Pathways for Compute Governance Technical and policy pathways exist to increase oversight and monitoring for supercomputers powered by thousands of high-end AI chips. These notional pathways may also open the door to a broader governance regime – beyond the October 7, 2022 export controls – that could restrict malign uses of generative AI, such as disinformation or WMD development, and enable attribution or responses after an incident occurs. Options could include:41 Leverage U.S. leadership in cloud services to cut off access to clear and present national security threats. If U.S. firms were to retain leadership in providing cloud access to high-end AI chips, U.S. regulators might have the ability to ask firms to “pull the plug” on models that are used in malign ways, such as running disinformation campaigns or designing weapons of mass destruction. Create a registry for large compute clusters. Under such a regime, actors that possess large supercomputers for AI training and inference would be required to register with a central authority, which could monitor compliance for high-risk use cases. Deploy hardware-enabled mechanisms for traceability or responsible use. It is technically feasible to implant unique identifiers in cutting-edge AI chips or server modules that could function, in theory, as a cyber-physical “fingerprint.” If robustly developed, such a program could help enhance supply chain traceability and responsible use.42 |
Energy & Sustainability
Continued compute scaling for generative AI is unsustainable at today’s rate, both in terms of environmental impact and cost. Training frontier large language models generates massive amounts of carbon emissions while consuming significant water resources.43 Likewise, if compute costs were to continue rising at the same pace, the cost to train the world’s largest AI model would approach the annual cost of the Apollo Program within a decade and exceed U.S. GDP by 2036.44
Solving this problem will require firms to build more efficient algorithms and hardware that require less compute and energy resources. AI firms can make their models more energy efficient by removing unnecessary layers, a technique known as “pruning,” or by streamlining neural network architectures.45 These techniques can improve energy efficiency by four to 10 times or more and, when combined with specialized hardware accelerators, energy efficiency can potentially be improved by several orders of magnitude.46 Models can also be re-used or optimized for certain tasks, reducing the need for more energy-intensive and costly training runs.47
Key Actions
In the near term, the White House should launch a Green AI Initiative with allies and partners.48 Such an initiative would consult with industry experts and researchers and share best practices for minimizing the carbon footprint associated with AI training and inference.49 The program could be coordinated by the National Artificial Intelligence Initiative Office within the Office of Science and Technology Policy (OSTP) using existing resources.50 Sustainable AI also represents a promising area of collaboration for groupings such as the Quad51 or the U.S.-EU Trade and Technology Council (TTC), which could develop agreements on sustainable AI standards.
Congress should encourage firms to disclose energy usage and carbon emissions from AI training.52 Additional transparency can provide an incentive to reduce the amount of energy used for model training and inference. Transparency should also extend to whether models are powered using clean energy sources. Congress could include these disclosure requirements in AI legislation.53
Novel Hardware Paradigms
To make matters worse, booming compute demand from GenAI comes at a time when chip manufacturing is running up against the laws of physics. The world’s most advanced chips feature patterns just 3 nanometers across, or roughly 25,000 times smaller than the width of a human hair.54 As today’s chip manufacturing techniques push up against physical limits, Moore’s Law – the prediction that the amount of computing power on a chip will double every two years – is slowing down.55
Several pathways exist to meet this challenge. The first is building specialized chips and systems for AI workloads. Over the past few years, for example, AI firms have moved away from using general-purpose chips to train models and instead rely on Graphical Processing Units (GPUs) that can perform many computations in parallel. Building new kinds of specialized AI accelerators, which match custom hardware for specific applications, holds tremendous promise. U.S.-based large technology firms and AI chip startups are moving to capitalize on this trend, suggesting that substantial market incentives already exist.56
New computing paradigms like quantum and neuromorphic computing are well into development worldwide to provide compute gains beyond Moore’s Law. The chip industry is already pursuing 3D heterogeneous integration – scaling Moore’s Law in the third dimension by stacking components and connecting them in new ways.57 DARPA’s Electronics Resurgence Initiative 2.0 is pursuing a robust research program in this area, with a focus on driving rapid commercialization while supporting defense mission requirements.58 But a number of other emerging paradigms exist that could help meet skyrocketing compute demand in the intermediate term: examples include silicon photonics; heterogeneous classical-quantum computing; neuromorphic and in-memory computing; and superconducting electronics.59 Today, many of these paradigms face hurdles to commercialization, making them ideal targets for government investment and other policies to de-risk private investment.60
Key Action
Launch moonshot projects focused on incentivizing investments in emerging microelectronics paradigms that could power future generations of AI models. True microelectronics moonshots can occur across the U.S. innovation ecosystem, ranging from companies to DARPA’s Microsystems Technology Office. But who sets the bar for the nation, supports implementation, and reports results to the President? Short of a Technology Competitiveness Council,61 the National Semiconductor Technology Center (NSTC), already funded under the CHIPS and Science Act and scheduled to launch later this year, could be the place. To achieve genuine moonshots, a coordinating mechanism across government programs is needed.62 In addition, the NSTC Investment Fund should be rapidly stood up and sufficiently resourced with at least $1 billion in government seed funding. As part of its mission, the NSTC has an opportunity to serve as an incubator, providing a one-stop-shop “help desk” for innovators as they attempt to navigate the public-private ecosystem.
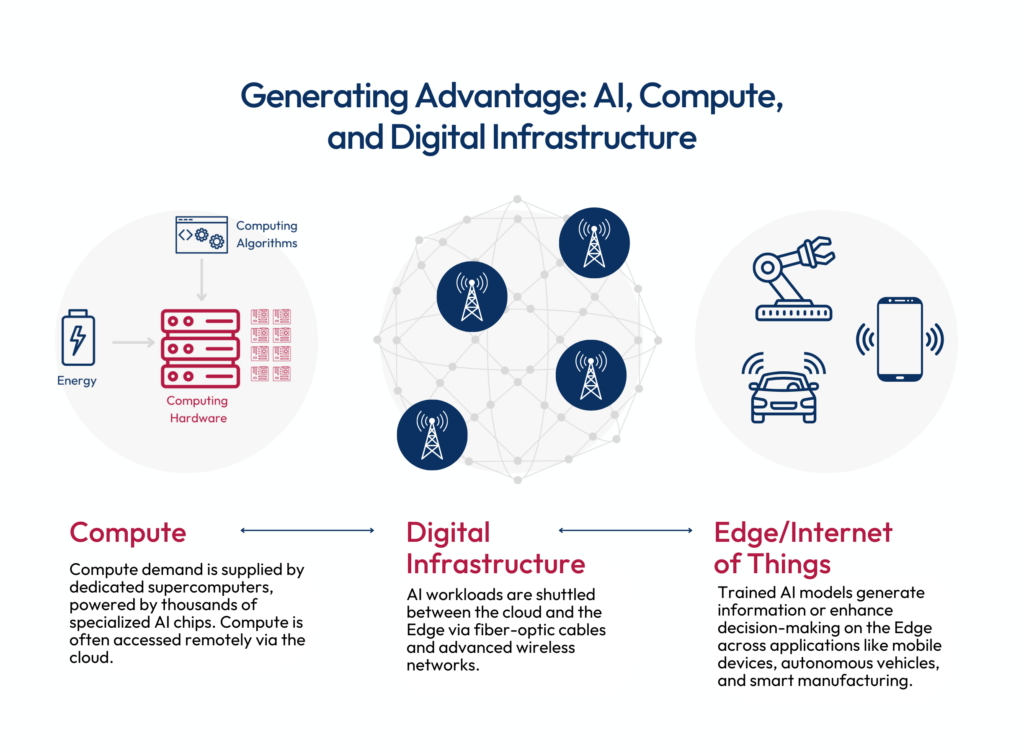
Data
Data, plus the infrastructure needed to query, process, and transport it to and from connected devices and users, is essential to AI leadership. GenAI models require huge amounts of data for training: OpenAI’s GPT-3 is estimated to be trained on 45 terabytes of text data – equivalent to a quarter of the entire Library of Congress – at an estimated cost of several million dollars.63 To maintain our competitive advantage in GenAI, the United States must treat the nation’s data as a strategic asset by organizing coherent approaches to digital infrastructure and data governance.
Digital Infrastructure
Digital infrastructure, from fiber optic cables and infrastructure for wireless networks to data centers and satellite arrays, is the backbone for incorporating AI in the cloud and on edge devices. But delivering useful AI-powered applications – from smart cities and smart factories to autonomous vehicles – requires a unified national approach. The United States must develop a national strategy for strengthening its digital infrastructure, including by ensuring U.S. leadership in advanced networks which store, process, and transport data nationwide.64 Internationally, the United States must also strengthen coordination with allies and partners on standards, which will shape future leadership in networks and AI.65
Key Actions
Develop a National Strategy for Digital Infrastructure. Congress has appropriated more than $100 billion for digital infrastructure since 2018, but the United States lacks a coordinated, strategic approach to strengthen America’s digital backbone.66 In particular, the United States must position itself to win the competition for the next generation of advanced networks by prioritizing industrial and enterprise use-cases.67 Wireless networks will be crucial as the proliferation of GenAI-powered applications increases data flows at the edge. Congress should require the Federal Communications Commission, in coordination with the Departments of Commerce and Agriculture and other relevant agencies, to deliver a National Digital Infrastructure Strategy within 270 days.68
Assert U.S. leadership in international network technology standards-setting bodies. The PRC has organized its firms to show up in force in these bodies and advance its political objectives. The National Institute of Standards and Technology (NIST), working with others like the Department of State, should develop a clear strategy for 6G and forthcoming network standards to help support, not replace, the industry-led standards-setting process.69 The United States should coordinate these efforts with democratic allies and partners,70 including by establishing a “Free 6G Leadership Group.”
Free 6G Leadership Group In concert with private sector-led efforts like the NextG Alliance,71 the U.S. government and other advanced democracies should form a group to support proactive leadership in development of 6G network standards and principles in line with democratic values. U.S. and European governments have begun discussing 6G within the U.S.-EU Trade and Technology Council (TTC).72 These stakeholders should expand engagements to include Japan, Australia, and India – which have created an Open RAN-focused working group within the Quad – and other partner countries.73 |
Catalyze domestic and friendshored production of key network components. To ensure stable and trusted supply chains, Congress and the Department of Commerce should leverage incentives, R&D funding, and further restrictions on PRC-made components to promote the production of trustworthy alternatives to PRC core, RAN, Internet of Things (IoT), and satellite components.74
Data Governance
The United States has long enjoyed significant advantages in data access for AI training – owing to the global presence of U.S. tech firms and large volumes of English language text on the Internet – but its current ad hoc approach to data governance is putting U.S. leadership at risk.75 America is home to the world’s largest tech companies, has the most data centers, and leads in the big data market.76 But China is making gains with a comprehensive digital strategy that restricts access to its large domestic data resources and leverages its tech firms’ growing global footprint to access global data resources.77 Meanwhile, the EU is adopting a regulation-first approach that prioritizes privacy but threatens to dampen innovation and limit opportunities for U.S. firms.78
The United States cannot afford to take a wait-and-see approach, sitting on the sidelines as others write the rules for its digital future and waiting for its adversaries to stumble.79 The United States must resolve uncertainty around regulatory issues like data privacy and ownership, make valuable government data more accessible, and create public-private partnerships to aggregate siloed government, private sector, and academic datasets for strategic effect.80 In addition to facilitating public-private partnerships for sharing data, these partnerships should be leveraged to create unique data sets that can be treated as “national assets” (e.g., shared medical data sets to support improved diagnosis and therapies, and information for the U.S. workforce about shifting supply and demand for different types of jobs and skills and associated opportunities for continuing education).
Key Actions
Pass comprehensive data privacy legislation. As AI permeates industries, a data governance model will be instrumental in efforts to establish checks and balances that ensure data and technology are used ethically, safely, and in keeping with our nation’s values. Legislation is a key element of such a model and is urgently needed to unify the patchwork of state laws and enable trust in the U.S. data ecosystem to set conditions for new digital trade agreements.81 By defining legal requirements surrounding data usage, the United States can maximize data as a strategic asset while protecting rights such as privacy and addressing harmful society-level impacts.82
Pave the way for digital trade in the age of generative AI. The AI revolution dramatically raises the stakes for us to work with democratic allies and partners to increase digital trade. In particular, firms and workers in the United States and the EU, two of the largest markets in the world, stand to gain economically by enabling cross-border data flows and reducing costs associated with customs duties on digital transactions.83 The Office of the United States Trade Representative (USTR), in coordination with the Departments of Commerce and State, must accelerate efforts to develop compatible approaches to AI and data flows and regulation with the EU and other democracies.84
Explore the need for intellectual property rights in data. The Secretary of Commerce, in coordination with the United States Patent & Trademark Office and other relevant agencies, should explore the implications of intellectual property rights in data for national security, economic, and privacy issues, especially as pertaining to domestic and international data sharing between private and public entities.85
People
People are America’s most important asset for AI competitiveness. Leadership in generative AI requires the ability to attract and retain the “rare talent to make AI breakthroughs” – the “holy grail” for enhancing U.S. national competitiveness.86 While the United States remains an attractive destination for technical experts, increasing numbers of high-skilled immigrants are choosing to take their talents elsewhere due to the administrative burdens of the immigration system.87 In addition, providing high quality STEM education and pathways for reskilling and training are vital to unlock productivity gains and create opportunities for the American workforce. Training skilled end-users is a critical factor in the diffusion of any general-purpose technology.88
Immigration
Generative AI opens up a new world for employment, skills, and innovation capacity, but its potential benefits will only materialize if the United States continues to attract the world’s top AI talent. Sixty-five percent of America’s top AI companies have immigrant founders or co-founders.89 Despite its current position of leadership, the United States faces an increasingly troubling tech talent deficit that must be overcome if it is to realize potential GenAI-enabled productivity gains.90 High-skilled immigrants play a crucial role in alleviating this deficit, and the current immigration system’s hurdles not only prevent talent from coming to the United States, but inadvertently direct talent elsewhere.91
The United States must act quickly to create new pathways to attract AI talent from around the world. Failure to improve our system is prompting global talent to go elsewhere, harming America’s ability to innovate.92 Other countries are capitalizing on this trend: Canada, for example, implemented a new program in July 2023 to attract H1-B visa holders frustrated with the American system to work in Canada, with clear pathways to permanent residency.93 In addition, ex-China emigration, including technical experts, is rising, offering the United States an opportunity to attract AI talent.94
Key Actions
Increase the H-1B visa cap. A comprehensive strategy from the Departments of Labor and Homeland Security is required to improve the H-1B system and, in collaboration with Congress, recommend a cap increase to 195,000, as well as other measures to enable a greater inflow of vital technical and STEM talent.95
Grant conditional green cards to STEM Ph.D. graduates from accredited American universities. Congress should consider granting conditional green cards to STEM Ph.D. graduates from accredited American institutions, with an initial focus on students from partner nations, and also reconsider adding a new visa category for technology entrepreneurs.96
Adjust return-to-home requirements for J-1 visa holders. The Department of State should adjust return-to-home requirements for J-1 exchange visa holders working or studying fields pertaining to AI and other critical emerging technologies to reduce the need for such holders to return to their home nations for two years prior to returning to the United States.97
Workforce Development
The diffusion of GenAI could dramatically improve the nation’s productivity,98 but at this early stage, it remains unclear how widespread deployment of these capabilities will affect labor markets and the nature of work in the long run.99 To unlock new opportunities for the American workforce, economic policymakers should invest in and update worker training and development programs that are adaptable to the rapidly changing nature of GenAI tech.
Key Action
Form a National Commission on Automation and the Future of Work. Congress should form a bipartisan commission to review the current state of automation in the United States, analyze its impact on our workforce and economic competitiveness, and propose policy changes that address human-machine teaming, upskilling, and reskilling.100
STEM Education
GenAI holds enormous potential for improving the U.S. STEM education system. It can provide personalized learning for students while easing burdens placed on educators. That said, it is important to acknowledge that these systems could exacerbate the digital divide or problems associated with social media usage.
Key Action
Develop guidelines for deployment of GenAI in the classroom. NIST should coordinate with the Department of Education’s Office of Elementary and Secondary Education and state-level education agencies to ensure the prioritization – and appropriate use – of GenAI tools within the K-12 curriculum, developing a set of guiding principles in the process.101 In addition, Congress should increase grant funding from NSF by $25 million, in coordination with the Department of Education and non-profit and private sector organizations, to increase school systems’ access to the most updated large language models.102
Conclusion
The advent of generative AI marks an economic turning point. As with past general-purpose technologies, nations that lead in the adoption of generative AI will reap the long-term benefits of increased productivity and economic growth. Productivity gains mean additional resources for national priorities, from defense spending to R&D and social programs. GenAI also marks an opportunity to open digital markets among allies and partners who treat data as an asset that can be leveraged to enrich and empower rather than control and repress. Without a focused effort, however, continued leadership cannot be assured. By shoring up the essential building blocks for AI leadership, the United States can ensure democratic competitiveness in the Age of AI.
Endnotes
- For example, the PRC leads the world in 5G network hardware, hypersonic missiles, EV batteries, and (as of first quarter 2023) auto exports, and its social media apps have billions of users globally. See Harnessing the New Geometry of Innovation, Special Competitive Studies Project at 60-92 (2022); David Lin, et al., China in the Drivers’ Seat, Special Competitive Studies Project (2023). In 2017, the PRC issued a plan to lead the world in AI by 2030 and it is rapidly automating its manufacturing industry. Pablo Robles, China Plans to Be a World Leader in Artificial Intelligence by 2030, South China Morning Post (2018); Mai Tao, China Aims for Global Leadership in Robotics with New Five-Year Plan, Robotics & Automation News (2022).
- Top 10 Emerging Technologies of 2023, World Economic Forum and Frontiers Media (2023); Alistair Dieppe, Global Productivity: Trends, Drivers, and Policies, The World Bank (2021); Christopher Pissarides, et al., Measuring the Welfare Effects of AI and Automation, The Centre for Economic Policy Research (2019).
- For example, digitally-delivered services accounted for 54 percent of total global services exports in 2022. Jordan G. Heiber, Why Digital Trade Is Critical to the U.S. and Global Economies, U.S. Chamber of Commerce (2023).
- Robert D. Atkinson, Why Federal R&D Policy Needs to Prioritize Productivity to Drive Growth and Reduce the Debt-To-GDP Ratio, Information Technology and Innovation Foundation (2019).
- Erik Brynjolfsson & Lorin M. Hitt, Beyond Computation: Information Technology, Organizational Transformation and Business Performance, Journal of Economic Perspectives (2000); Richard G. Lipsey, et al., Economic Transformations: General Purpose Technologies and Long-Term Economic Growth, Oxford University Press (2005); Timothy F. Bresnahan & Manuel Trajtenberg, General Purpose Technologies: “Engines of Growth?”, National Bureau of Economic Research at 18-21 (1992); Erik Brynjolfsson, et al., The Productivity J-Curve: How Intangibles Complement General Purpose Technologies, American Economic Journal: Macroeconomics (2021).
- OpenAI’s multimodal ChatGPT, for instance, acquired 100 million users just two months after its release, making it the fastest growing consumer application in history. See Andrew R. Chow, How ChatGPT Managed to Grow Faster than TikTok or Instagram, Time (2023).
- Michael Chui, et al., The Economic Potential of Generative AI: The Next Productivity Frontier, McKinsey & Company (2023). Germany’s GDP in 2022 was $4.07 trillion. See GDP (current US$) – Germany, The World Bank (last accessed 2023).
- Goldman Sachs estimates that GenAI could drive a $7 trillion increase in annual global GDP over 10 years, increasing productivity growth by 1.5 percentage points in the same period. Bloomberg Intelligence expects an “explosion of growth” in the GenAI software sector, with revenues rising to $1.3 trillion by 2032 (from $40 billion in 2022). Analysts also claim that the use of GenAI-powered coding assistance applications alone could “boost global GDP by over $1.5 trillion by 2032.” See The Potentially Large Effects of Artificial Intelligence on Economic Growth, Goldman Sachs (2023); Jake Rudnitsky, ChatGPT to Fuel $1.3 Trillion AI Market by 2032, New Report Says, Bloomberg (2023); Sida Peng, et al., The Impact of AI on Developer Productivity: Evidence from GitHub Copilot, arXiv (2023).
- Yiwen Lu, As Businesses Clamor for Workplace A.I., Tech Companies Rush to Provide It, New York Times (2023); Hugh Son, Morgan Stanley Is Testing an OpenAI-Powered Chatbot for Its 16,000 Financial Advisors, CNBC (2023); The Future of Generative AI for Legal and Tax Professionals, Axios (last accessed 2023); Michael Sentonas, CrowdStrike Introduces Charlotte AI, Generative AI Security Analyst, CrowdStrike (last accessed 2023).
- Erik Brynjolfsson, et al., Generative AI at Work, National Bureau of Economic Research (2023).
- Shakked Noy & Whitney Zhang, Experimental Evidence on the Productivity Effects of Generative AI, Science (2023).
- Eric Schmidt, This Is How AI Will Transform the Way Science Gets Done, MIT Technology Review (2023).
- Brent Mitchell, How AI Can Enhance Government Services, Improve Productivity, and Better Support Communities, Google Cloud Blog (2023); Christina Montgomery, Automation With a Human Touch: How AI Can Revolutionize Our Government, U.S. Chamber of Commerce (2023); Alan Holden, et al., Designing for the Public Sector with Generative AI, Deloitte (2023); Generative AI for U.S. Federal Agencies, Accenture (last accessed 2023).
- Seth Colaner, How the U.S. Patent Office Is Keeping up with AI, VentureBeat (2020); Andrew Toole, et al., The Promise of Machine Learning for Patent Landscaping, Santa Clara High Technology Law Journal (2020). Likewise, the Department of State is considering how to use GenAI to improve contract writing, market research, and acquisition planning, while members of Congress have begun experimenting with GenAI to produce constituent response drafts, policy papers, and bills. See Billy Mitchell, State Department Considers Generative AI for Contract Writing, FedScoop (2023); Nihal Krishan, Congress Gets 40 ChatGPT plus Licenses to Start Experimenting with Generative AI, FedScoop (2023).
- Researchers claim, for example, that “around 80% of the U.S. workforce could have at least 10 percent of their work tasks affected” by GenAI. See Tyna Eloundou, et al., GPTs are GPTs: An Early Look at the Labor Market Impact Potential of Large Language Models, arXiv (2023). Amelia Sandhovel, Crafting the Future of Work: A Policy Framework for the Automation Age, Economic Innovation Group (2023).
- David Lin & Katie Stolarczyk, Automation Across Industries: Industrial Automation and the American Economy, Special Competitive Studies Project (2023); Robert D. Atkinson, Robots, Automation, and Jobs: A Primer for Policymakers, Information Technology and Innovation Foundation (2017); Ajay Agrawal, et al., Artificial Intelligence: The Ambiguous Labor Market Impact of Automating Prediction, The Journal of Economic Perspectives (2019); David H. Autor, Why Are There Still So Many Jobs? The History and Future of Workplace Automation, The Journal of Economic Perspectives (2015); Daron Acemoglu & Pascual Restrepo, Automation and New Tasks: How Technology Displaces and Reinstates Labor, The Journal of Economic Perspectives (2019).
- Erik Brynjolfsson, The Turing Trap: The Promise & Peril of Human-Like Artificial Intelligence, Daedalus (2022); Emma Goldberg, A.I.’s Threat to Jobs Prompts Question of Who Protects Workers, New York Times (2023).
- Jan Hatzius, et al., The Potentially Large Effects of Artificial Intelligence on Economic Growth, Goldman Sachs (2023).
- Martin Neil Baily, et al., Machines of Mind: The Case for an AI-Powered Productivity Boom, Brookings Institution (2023).
- Stephanie Ferguson, Understanding America’s Labor Shortage: The Most Impacted Industries, U.S. Chamber of Commerce (2023).
- See Final Report, National Security Commission on Artificial Intelligence at 2-4 (2021); Micah Musser, et al., “The Main Resource is the Human”: A Survey of AI Researchers on the Importance of Compute, Center for Security and Emerging Technology (2023).
- Jaime Sevilla, et al., Compute Trends Across Three Eras of Machine Learning, EpochAI (2022); Lennart Heim, Compute Trends Across Three Eras of Machine Learning, Montreal AI Ethics Institute (2023).
- Guido Appenzeller, et al., Navigating the High Cost of AI Compute, Andreessen Horowitz (2023).
- Jaime Sevilla, et al., Compute Trends Across Three Eras of Machine Learning, EpochAI (2022); Charlie Giattino, Artificial Intelligence, Our World in Data (last accessed 2023).
- Stephen Nellis, Nvidia Chips Away at Intel, AMD Turf in Supercomputers, Reuters (2023).
- Commerce Implements New Export Controls on Advanced Computing and Semiconductor Manufacturing Items to the People’s Republic of China (PRC), U.S. Department of Commerce, Bureau of Industry and Security (2022). To complement the export controls, on August 9, 2023, the President issued an Executive Order that included new prohibitions and notification requirements on certain U.S. outbound investments in countries of concern in national security technologies including microelectronics. The new measures are intended to impose further limits on the transfer of expertise from U.S. investors to entities in China, potentially hampering China’s ability to develop export-controlled technologies indigenously. See Executive Order on Addressing United States Investments in Certain National Security Technologies and Products in Countries of Concern, The White House (2023).
- John VerWey, The Other Artificial Intelligence Hardware Problem, Computer (2022).
- Strengthening and Democratizing the U.S. Artificial Intelligence Innovation Ecosystem: An Implementation Plan for a National Artificial Intelligence Research Resource, National Artificial Intelligence Research Resource Task Force (2023); Liza Tobin, et al., Building a National Delivery System for Data: Taking Stock of China’s 5G, Broadband, and Data Center Buildout, Special Competitive Studies Project (2023).
- American cloud providers rent access to GPU clusters at a rate of $1.00 to $4.00 per chip. Guido Appenzeller, et al., Navigating the High Cost of AI Compute, Andreessen Horowitz (2023).
- Felix Richter, Big Three Dominate the Global Cloud Market, Statista (2023).
- David McCabe, China’s Cloud Computing Firms Raise Concern for U.S., New York Times (2023); Simon Sharwood, Oracle and Huawei Clouds the Big Movers on Gartner’s Conjured Quadrilateral, The Register (2022).
- Rama Elluru, et al., Crossing the Digital Atlantic: A U.S.-EU Agenda for the Age of AI, Special Competitive Studies Project (2023).
- Felix Richter, Big Three Dominate the Global Cloud Market, Statista (2023).
- See, e.g., Haomiao Huang, The Generative AI Revolution Has Begun – How Did We Get Here?, ArsTechnica (2023). Final Report, National Security Commission on Artificial Intelligence at 440-441 (2021).
- Final Report, National Security Commission on Artificial Intelligence at 440-441 (2021); Strengthening and Democratizing the U.S. Artificial Intelligence Innovation Ecosystem: An Implementation Plan for a National Artificial Intelligence Research Resource, National Artificial Intelligence Research Resource Task Force (2023).
- See Press Release, AI Caucus Leaders Introduce Bipartisan Bill to Expand Access to AI Research (2023).
- Strengthening and Democratizing the U.S. Artificial Intelligence Innovation Ecosystem: An Implementation Plan for a National Artificial Intelligence Research Resource, National Artificial Intelligence Research Resource Task Force (2023).
- See e.g., Eleanor Olcott, et al., Chinese AI Groups Use Cloud Services to Evade US Chip Export Controls, Financial Times (2023).
- Executive Order on Taking Additional Steps to Address the National Emergency with Respect to Significant Malicious Cyber-Enabled Activities, The White House (2021).
- Tim Fist, et al., Chinese Firms Are Evading Chip Controls, Foreign Policy (2023).
- For additional discussion of these options, see Robert Wiblin & Keiran Harris, Lennart Heim on the Compute Governance Era and What Has to Come After, 80,000 Hours (2023).
- For an example of a compute governance regime based on hardware-enabled mechanisms, see Yonadav Shavit, What Does It Take to Catch a Chinchilla? Verifying Rules on Large-Scale Neural Network Training via Compute Monitoring, ArXiv (2023). Tim Fist, et al., Chinese Firms Are Evading Chip Controls, Foreign Policy (2023).
- Measuring the Environmental Impacts of Artificial Intelligence Compute and Applications: The AI Footprint, OECD (2022); Pengfei Lei, et al., Making AI Less “Thirsty”: Uncovering and Addressing the Secret Water Footprint of AI Models, ArXiv (2023); Payal Dhar, The Carbon Impact of Artificial Intelligence, Nature Machine Intelligence (2020).
- Andrew J. Lohn & Micah Musser, AI and Compute: How Much Longer Can Computing Power Drive Artificial Intelligence Progress?, Center for Security and Emerging Technology (2022).
- Kavitha Prasad, Achieving a Sustainable Future for AI, MIT Technology Review (2023); Vivian Sze, Energy-Efficient Deep Neural Networks, MIT Energy Efficient Multimedia Systems Group (2023).
- Vivian Sze, Energy-Efficient Deep Neural Networks, MIT Energy Efficient Multimedia Systems Group (2023); Charles E. Leiserson, et al., There’s Plenty of Room at the Top: What Will Drive Computer Performance After Moore’s Law?, Science (2020).
- Kavitha Prasad, Achieving a Sustainable Future for AI, MIT Technology Review (2023).
- Roberto Verdecchia, et al., A Systematic Review of Green AI, ArXiv (2023).
- See, e.g., David Patterson, Carbon Emissions and Large Neural Network Training, ArXiv (2021); Kavitha Prasad, Achieving a Sustainable Future for AI, MIT Technology Review (2023).
- About the NAIIO, National Artificial Intelligence Initiative Office (last accessed 2023).
- The Quad includes Japan, Australia, India, and the United States.
- Such a rule has been included in a recent version of the EU AI Act. See P9_TA(2023)0236, Artificial Intelligence Act, European Parliament (2023).
- See e.g., SAFE Innovation Framework, Office of Sen. Majority Leader Chuck Schumer (2023).
- Understanding Nanoscale | Part 1: How Small Is Small?, Medium (2020).
- See John Shalf, The Future of Computing Beyond Moore’s Law, Philosophical Transactions of the Royal Society (2020).
- What Is an AI Accelerator?, Synopsys (last accessed 2023); Doug O’Laughlin, The Coming Wave of AI, and How Nvidia Dominates, Fabricated Knowledge (2023).
- What Is 3DIC Technology?, Synopsys (last accessed 2023).
- Electronics Resurgence Initiative 2.0, DARPA (last accessed 2023).
- In-memory computing, for example, improves energy efficiency by placing processing and memory units closer together, allowing for increasingly brain-like neuromorphic computing. Silicon photonics uses light to replace the wires in and between chips. Superconducting circuits drop the level of resistance in a circuit to zero, allowing for extremely fast, energy-efficient computation. See Chapter 16: Emerging Research Devices, Heterogeneous Integration Roadmap: 2023 Edition (2023); National Action Plan for U.S. Competitiveness in Microelectronics, Special Competitive Studies Project (forthcoming).
- On government de-risking, see Restoring the Sources of Techno-Economic Advantage, Special Competitive Studies Project at 15-16 (2022). Enhancing the United States’ R&D tax credit – which lags significantly behind other industrialized nations – would also crowd in industry R&D dollars, in the microelectronics industry and elsewhere. Robert D. Atkinson, The Case for Improving America’s Research and Experimentation Tax Credit, The Hill (2021).
- In 2022, Congress considered legislation for both a Technology Competitiveness Council (TCC) and Office of Global Competition Analysis (OCA). H.R. 8027, To Establish within the Executive Office of the President a Technology Competitiveness Council (2022); Courtney Albon, Lawmakers Propose ‘Technology Competitiveness Council’ to Champion US Innovation, C4ISRNet (2022); S. 4368, American Technology Leadership Act of 2022 (2022); Daniel Flatley, Senators Wary of China’s Tech Prowess Seek Competition Office, Bloomberg (2022). More recently, in 2023, The U.S. Senate has also introduced a legislative bill to establish an OCA. S.1873 – Global Technology Leadership Act (2023).
- Ana Swanson & Don Clark, Commerce Dept. Outlines Plans to Fund Cutting-Edge Chip Research, New York Times (2023).
- What Is Generative AI?, McKinsey & Company (2023).
- The most recent comprehensive U.S. digital infrastructure plan was produced by the FCC in 2010. See Connecting America: The National Broadband Plan, Federal Communications Commission (2010). After more than a decade, a 2022 study by the U.S. Government Accountability Office found that federal broadband efforts are fragmented and overlapping and recommended the President develop a national broadband strategy. Broadband: National Strategy Needed to Guide Federal Efforts to Reduce Digital Divide, Government Accountability Office (2022).
- Robert D. Atkinson & Martijn Rasser, Help US Companies Compete Against China on Technology Standards, RealClear Policy (2022).
- Restoring the Sources of Techno-Economic Advantage, Special Competitive Studies Project at 32 (2022).
- SCSP has proposed a plan to do this. See National Action Plan for U.S. Leadership in Advanced Networks, Special Competitive Studies Project (2023).
- The Department of Agriculture manages broadband programs for rural areas, schools, and libraries. For more on a proposed national strategy, see National Action Plan for U.S. Leadership in Advanced Networks, Special Competitive Studies Project, at 6-7 (2023).
- Including those developed by the 3rd Generation Partnership Project (3GPP), an international body focused on technical specifications for mobile networks. Introducing 3GPP, 3GPP (last accessed 2023).
- Existing groupings such as the Quad (Australia, India, Japan, United States) and the TTC could be used.
- See NextG Alliance (2023).
- U.S.-EU Joint Statement of the Trade and Technology Council, The White House (2023); 6G Outlook, The European Commission (2023).
- See National Action Plan for U.S. Leadership in Advanced Networks, Special Competitive Studies Project at 17-18 (2023).
- National Action Plan for U.S. Leadership in Advanced Networks, Special Competitive Studies Project (2023) at 9-11.
- The United States has no comprehensive national data privacy standard; has valuable, but difficult to access and use, data across the US government; and no scalable model for aggregating public and private data, including insufficient infrastructure for data sharing, such as a national AI research cloud. See National Data Action Plan, Special Competitive Studies Project at 2 (2022); First Quarter Recommendations Memorandum, National Security Commission on Artificial Intelligence (2020); Strengthening and Democratizing the U.S. Artificial Intelligence Innovation Ecosystem: An Implementation Plan for a National Artificial Intelligence Research Resource, National Artificial Intelligence Research Resource Task Force (2023).
- Jonathan Ponciano, The World’s Largest Technology Companies In 2023: A New Leader Emerges, Forbes (2023); National Data Action Plan, Special Competitive Studies Project at 2 (2022).
- Emily de la Bruyere, et al., China’s Digital Ambitions: A Global Strategy to Supplant the Liberal Order, National Bureau of Asian Research (2022).
- See Rama Elluru, et al., Crossing the Digital Atlantic: A U.S.-EU Agenda for the Age of AI, Special Competitive Studies Project (2023).
- Beijing’s increasingly stringent measures to wall off foreign access to Chinese data is causing foreign businesses to rethink operations in China. See e.g., The Crackdown on Foreign Firms Will Deter Global Businesses – and Undermine China’s Own Interests, The Economist (2023).
- See National Data Action Plan, Special Competitive Studies Project (2022).
- For proposed principles for federal privacy legislation, see National Data Action Plan, Special Competitive Studies Project (2022). An American data governance model would also include additional regulatory and non-regulatory elements to protect privacy and promote accessibility of government and non-government data.
- Kevin Roose, A.I. Poses ‘Risk of Extinction,’ Industry Leaders Warn, New York Times (2023); Cade Metz & Gregory Schmidt, Elon Musk and Others Call for Pause on A.I., Citing ‘Profound Risks to Society’, New York Times (2023).
- The Digital Trade Revolution: How U.S. Workers and Companies Can Benefit from a Digital Trade Agreement, The U.S. Chamber of Commerce (2022). Additional agreements could be modeled after digital trade agreements with Japan and the digital chapter of the U.S.-Mexico-Canada Agreement (USMCA). See FACT SHEET on U.S.-Japan Digital Trade Agreement, U.S. Trade Representative (2019); United States-Mexico-Canada Agreement, U.S. Trade Representative (last accessed 2023).
- See Rama Elluru, et al., Crossing the Digital Atlantic: A U.S.-EU Agenda for the Age of AI, Special Competitive Studies Project (2023). Japan’s “Digital Free Flow with Trust” concept, launched at the G7 in 2023, offers principles for greater allied and partner collaboration on data governance. See Operationalizing Data Free Flow with Trust (DFFT), Center for Strategic and International Studies (2023).
- Testimony of Rama Elluru before the U.S. Senate Judiciary Subcommittee on Intellectual Property, Artificial Intelligence and Intellectual Property – Part I: Patents, Innovation, and Competition (2023).
- Final Report, National Security Commission on Artificial Intelligence at 2 (2021).
- Jon Marcus, With New ‘Talent Visas,’ Other Countries Lure Workers Trained at U.S. Universities, The Hechinger Report (2023).
- Jeffrey Ding, The Rise and Fall of Great Technologies and Powers, GitHub (2021).
- Ashley Gold, Exclusive: Immigrants Play Outsized Role in the AI Game, Axios (2023).
- Global and U.S.Talent Shortage Report – Statistics and Studies, Advantis Global (2023).
- Immigration Policy is Innovation Policy, Economic Innovation Group (last accessed 2023).
- Steve Case, Opinion: America’s Big Mistake in the War for High-Tech Talent, CNN (2023).
- Michelle Hackman & Paul Vieria, Canada Woos American H1-B Visa Holders Fed Up with U.S. Immigration System, The Wall Street Journal (2023).
- Nathaniel Taplin, China’s Brain Drain Threatens its Future, Wall Street Journal (2023). Among the emigrants are AI researchers leaving China due to U.S. export controls on high-end AI chips. Eleanor Olcott, et al., Microsoft to Move Top AI Experts From China to New Lab in Canada, Financial Times (2023).
- Final Report, National Security Commission on Artificial Intelligence at 424-425 (2021).
- International students play a vital role in advancing the innovation ecosystem. Roughly 40 percent of all STEM PhDs from American universities between 2000 and 2019 were international students, Jack Corrigan, et al., The Long-Term Stay Rates of International STEM PhD Graduates, Center for Security and Emerging Technologies (2022).
- J-1 visas are granted to students, professors, researchers, and specialists. This includes those studying and specializing in AI fields. See Exchange Visitors, U.S. Citizenship and Immigration Services (last accessed 2023); Eric Schmidt, To Compete With China on Tech, America Needs to Fix Its Immigration System, Foreign Affairs (2023).
- Michael Chui, et al., The Economic Potential of Generative AI: The Next Productivity Frontier, McKinsey & Company (2023); Generative AI Could Raise Global GDP by 7%, Goldman Sachs (2023); Erik Brynjolfsson, et al., Generative AI at Work, National Bureau of Economic Research (2023).
- Michael Chui, et al., The Economic Potential of Generative AI: The Next Productivity Frontier, McKinsey & Company (2023).
- The Commission could include the National Science Foundation, Departments of Labor, Commerce, and Education, General Services Administration, and others, and representatives from academia, labor unions, and manufacturing and technology sectors. Initiatives such as MIT’s Work of the Future have conducted research on a number of these objectives. See MIT Work of the Future, Massachusetts Institute of Technology (last accessed 2023); Kenan Fikri, Towards a More Socially and Spatially Inclusive Innovation Economy, Brookfield Institute (2019). Congress should appropriate approximately $15-20 million for this commission, based on previous commission budgets and factoring in inflation.
- Office of Secondary and Elementary Education, U.S. Department of Education (last accessed 2023).
- Figure based on National Science Foundation grants with similar efforts, which are between $20-$30 million for around 20-30 awards. See e.g., Computer Science for All (CSforAll: Research and RPPs), National Science Foundation (2023); Discovery Research PreK-12 (DRK-12), National Science Foundation (2023).