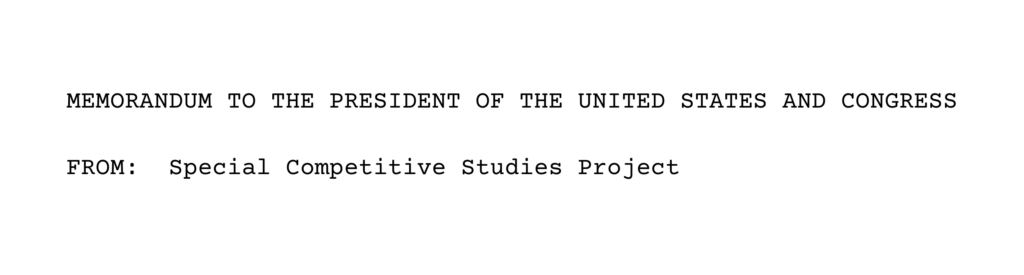
Introduction
The paramount determinant of success in the technology competition between the United States and China lies in each nation’s ability to wield innovation power at an unparalleled speed, unmatched quality, and unrivaled scale.1 System versus system, the security competition will come down to how technology diffuses into government affairs. That diffusion furthers a nation’s innovation power, from which it can build military capabilities, economic prosperity, and cultural influence around the world. The convergence of different technologies can yield a special kind of technology power that fundamentally alters national security architectures around the world.2 Historical convergence, like the combined use of the printing press and the compass, further encouraged nations to explore the world. The merging of electrification and mechanization paved the way for the Industrial Revolution.3 The proliferation of data as a result of the Internet Age has led to revolutions in digitization and helped spark the Age of AI, where vast quantities of data are created each day, converging with AI paradigms like machine learning, self-reflection,4 auto code-creation,5 and new forms of advanced modeling pushing new boundaries in technology innovation.
Generative artificial intelligence (GenAI) is converging with other fields that will both change the very nature of how we innovate and usher in a stronger, more general form of AI. Thus, mastering GenAI will be critical in technology competition and the United States should adopt measures to organize, drive, and ensure it has the fuel and resources to sustain America’s role as the world’s leading innovation power. It is only the beginning. In this memo, we first explore how GenAI is acting like an “innovation flywheel.” Then we will describe moves the nation could make to translate such innovation power into greater national security.
The GenAI Innovation Flywheel
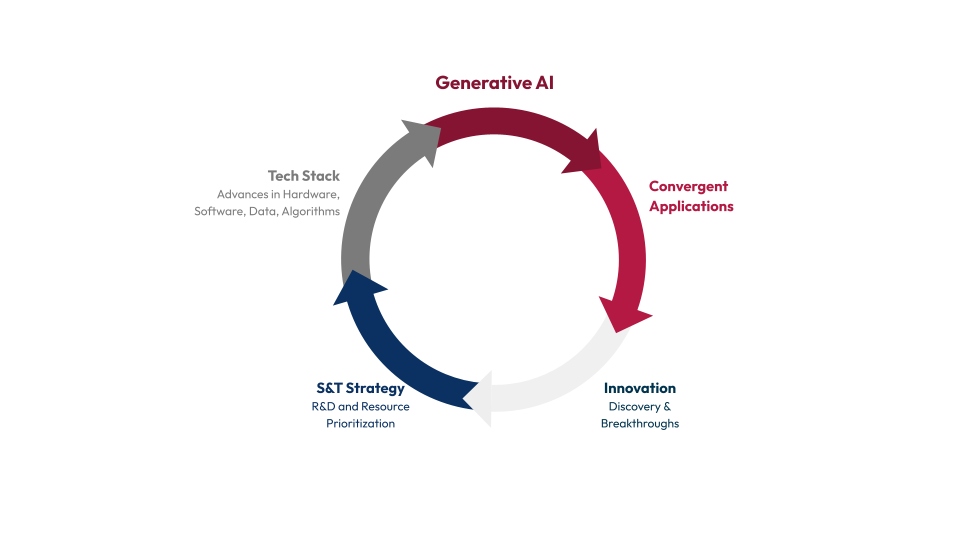
GenAI is driving a flywheel6 of value creation in which innovation will beget more, faster, and broader innovation. This will ultimately bring us closer to the prospect of a more general form of AI, spinning out many other technologies along the way.7 This flywheel occurs in four basic stages: first, GenAI is converging with other strategic technology sectors (biotech, energy, advanced manufacturing) to create an array of applications including many that cut across all fields (GenAI+). Second, the resulting innovations are changing the way the world works and helping people across society see the immense opportunity of AI.8 Third, these opportunities are driving organizations – from governments to startups – to reprioritize their strategies to adapt and leverage the value of GenAI.9 Finally, joining with independent compute innovation efforts already underway, such as quantum computing programs, this reprioritization fuels changes in the foundational technology inputs that make GenAI work: computing hardware, algorithms, data, and talent. This flywheel is already turning, and technological insights are likely to spin out at every stage. To be sure, there are potential limitations at each layer of the tech stack that could slow progress down. For example, we could run out of new data on which to train ever-larger models, and find synthetic data to be a poor substitute.10 But GenAI greatly expands our toolbox to anticipate and design out these obstacles, allowing progress to churn on.
GenAI Convergence with Strategic Tech Sectors
Some early rotations of this flywheel are driving new GenAI applications across strategic technology sectors, in turn boosting economic productivity.11 They are also enabling new pathways to scientific discovery itself, changing the way basic and applied R&D is being conducted, and more broadly, changing the way we innovate.12 In the longer term, these tools will help us answer some of the most vexing questions of the global technology competition: what are the next-generation battery chemistries that will underpin the world’s energy transition? What are the new active pharmaceutical ingredients (APIs) that will redefine the future of biotechnology and human life? To what degree will microelectronics system architectures improve when every designer has an AI co-pilot? The global race to find the answers has already begun, and the nation(s) able to innovate faster and better will have more power.
The step change that GenAI creates for innovation is multi-faceted. It is a natural language interface for machine intelligence, expanding humans’ ability to team up and interact with machines in increasingly complex ways. It is an orchestrator that can stitch together machine-machine interactions in new and richer ways. And it is an inventor, building on existing scientific databases to discover entirely new insights and ultimately execute the entire scientific process on its own. The ways GenAI is already accelerating science are astounding. In July of 2021, DeepMind published AlphaFold, an AI model for predicting the structure of a protein from its amino acid sequence; within one year, the tool was used to predict structures for nearly all cataloged proteins known to science.13 Less than a year later, the first entirely AI-discovered and AI-designed drug had begun phase 2 clinical trials, notably in China.14 Yet AlphaFold was only possible because of the existence of publicly available datasets on which the model was trained and refined.15 Replicating the AlphaFold story for other scientific fields is not out of the question, but will only be possible through concerted efforts to combine data that is currently scattered, proprietary, and/or limited.
Cross-Cutting GenAI Applications | Sector-Specific Examples | ||
1. ModSim & Planning: Improve design functions via modeling and simulation16 2. Optimization & Productivity: Make systems more efficient and effective17 3. S&T Discovery: Learn, reason with, and draw insights from data18 4. Data Analytics: Visualize vast amounts of data into charts, graphs, and tables quickly19 5. A Unique User Interface: Create personalized compute capabilities20 6. New Automation: Automate research and natural language processing21 7. Code Creation: Generate code for tailored algorithms22 8. Synthetic Data Production: Fabricate datasets for research, modeling, and development23 9. Grant Writing: Quickly raise financial support for innovation24 10. Content Creation: Convey complex information in digestible, compelling formats25 | Compute computer science, applied mathematics, electrical engineering | Dataflow-centric hardware architectures that create step-changes in AI performance26 | |
Networks electrical engineering, computer science | Simplify network planning, configuration, and optimization27 Verification of Web3 identities28 Automated cyber attack/defend with GenAI UI29 | ||
Biotech materials science,30 mechanical engineering, chemical engineering, biology, life sciences | Lab automation coupled with GenAI-based experiment design31 Protein prediction with exponentially more accuracy32 Novel drug discovery33 Personalized medical treatments and therapies through GenAI-augmented protein creation34 | ||
Energy Generation & Storage materials science, chemistry, chemical engineering | Aid in clean energy production by optimizing power plant operations to improve efficiency and to reduce emissions35 Automate operations within nuclear fusion reactors36 | ||
Smart / Advanced Manufacturing materials science, mechanical engineering | Strengthen bonding and adhesion in manufacturing37 Optimize the molecular structure of new materials38 Train smarter robots that could be used to increase automation efficiencies39 |
Eight National Moves to Match the GenAI Moment
The United States will be well-positioned to lead if we organize, set audacious tech goals, and resource the R&D needed to shore up the nation’s innovation power for the age of GenAI. Here are eight ways to do so.
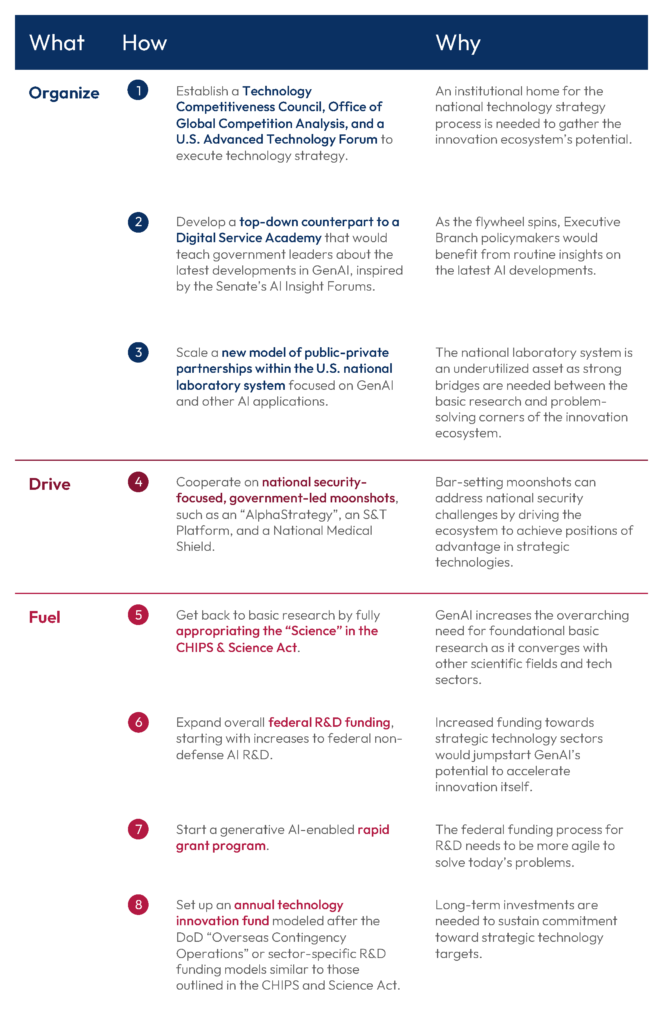
Organizing for Innovation Power
No one corner of the U.S. innovation ecosystem will be able to sustain long-term AI leadership alone. Advancing, diffusing, and harnessing this general purpose technology will require public-private efforts to organize national technology strategy and execute cross-cutting national innovation projects.
1. Establish a Small, Elite National Technology Strategy Organization
America needs an institutional home for the national technology strategy process to gather the innovation ecosystem’s potential. The United States has long embraced new organizational arrangements40 when the moment demands it.41 Today is one of those moments.
- The National Security Commission on Artificial Intelligence and SCSP have argued42 for a White House-based organization with two arms: an action-oriented Technology Competitiveness Council (TCC), and an analytic partner Office of Global Competition Analysis (OCA) that endures across administrations to provide long-term intellectual continuity on technology analysis and strategy.43 To meet this moment in technology innovation, SCSP recommended the establishment of a federally-chartered non-profit U.S. Advanced Technology Forum (USATF) that methodically convenes the private sector on a voluntary basis in support of the TCC and OCA. The combination of these three entities would transform the government’s innovation power if equipped with the authorities, resources, and expertise to execute a public-private national technology strategy method for the nation.44
2. Empower Policymakers to Get Smart on Tech
As the GenAI flywheel spins, even industry leaders are struggling to keep up with the rapidly changing applications and implications of this emerging technology. For government officials juggling competing policy priorities, the challenge of staying up-to-date on fast-moving technology developments is especially pronounced, particularly for those without deep technical backgrounds. On Capitol Hill, Senate Majority Leader Chuck Schumer is trying to address this through a series of AI Insight Forums, designed to educate Congressional leaders on the intricacies of AI as the legislative body considers regulations over the longer term.45 Some departments and agencies are also convening groups to assess GenAI for their specific mandates, such as the Department of Defense’s Task Force Lima.46 Yet Executive Branch leaders across the interagency would benefit from a similar routine mechanism at the interagency level for gathering insights on the latest AI developments.47
- Building on NSCAI’s calls to establish a Digital Service Academy48 – a new service academy that would take a bottom-up approach to train future civil servants in the digital skills required to modernize government – there needs to be a top-down counterpart aimed at keeping the U.S. government’s senior policymakers regularly updated on the latest emerging technology trends, to include GenAI. Taking inspiration from the Senate’s AI Insight Forums and nonprofit initiatives to bring together experts like SCSP’s Generative AI Task Force, the White House should convene the best AI minds in the country at regular intervals to teach government leaders about 1) the latest developments in GenAI and 2) how those might inform AI policies, adoption, and governance.
3. Unleash the National Lab Ecosystem with More Public-Private Partnerships
The vast national laboratory system49 is an underutilized asset in the American innovation ecosystem and should be leveraged to build new kinds of public-private partnerships. Expanding a reimagined model of public-private partnerships would allow the private sector increased access to the national labs’ latent resources, such as high-performance computing (HPC) capabilities, and augment the research teams working in line with the labs’ national missions. Currently, the Department of Energy (DOE) has difficulty scaling public-private partnerships due to funding constraints50 and the United States needs to support this work to maximize our ecosystem’s competitive advantages.
- The White House and Congress should cooperate to increase DOE’s ability to leverage public-private partnerships (PPPs) that advance AI and its convergence with other technology areas, and build lasting bridges between the basic research and problem-solving corners of the innovation ecosystem. This could come in the form of a new DOE directive for National Laboratories to assign a higher percentage of lab budgets toward creating PPPs focused on new approaches to GenAI and other AI applications for national security. One goal of this new lab-driven PPP model would be to grant industry — in particular startups — access to the labs’ latent resources such as HPC clusters. Conversely, the national labs would draw upon industry insights to advance their national security missions. PPPs of this ethos are already happening in the national labs — this would be scaling that trend.51
Driving Innovation, Shooting for the Moon
The U.S. government can achieve the unthinkable when the moment demands it. The Manhattan Project of the 1940s, the Apollo Program of the 1960s, and – most recently – Operation Warp Speed succeeded because the White House and Congress rose above all else to cooperatively muster the will and resources required to achieve those historic goals. If AI poses societal-scale risks on par with pandemics or nuclear war52 – not to mention immense opportunity – the United States needs to rediscover its sense of unified national purpose and urgency to drive next-generation innovation. The flywheel is accelerating, and global leadership cannot be left to chance.
4. Launch and Land Moonshots for the GenAI Era
The White House and Congress should cooperate on national security-focused, government-led moonshots with audacious and clear goals, leadership, and resourcing. As NSCAI identified in work that SCSP has continued, there are areas where bar-setting moonshots could address discrete challenges and opportunities by way of achieving positions of advantage in strategic technologies.
- Apply GenAI for National Security Decision Advantage: Like the modern corporation, the government with the best decision-making “factory”53 will have advantage over rivals. GenAI has demonstrated the technical pathway toward predictive decision making that could give nations real strategic advantages.54 Commercial players are already trying to build predictive analytic models for the U.S. government for specific domains,55 including one that predicted the start of the Russia-Ukraine War almost to the exact day.56 Technical, bureaucratic, and commercial hurdles make a whole-of-government approach unlikely to emerge absent national strategic direction and resources. The United States should adopt an “AlphaStrategy” approach — inspired by the AlphaGo model that beat the world’s best Go players57 — that stitches together predictive models across all domains58 to add a valuable voice in the room for national security decision-making at the highest levels.59
- Build an S&T Platform for Innovation Advantage: As a natural language interface for accessing complex information and processes at machine speed and scale,60 GenAI is poised to transform all stages of innovation, unlocking new capabilities and approaches for discovery, design, testing, building, and deployment.61 We will need new government R&D structures for an era in which science and technology are growing ever closer. Various efforts are already underway to use GenAI to accelerate R&D, yet such projects are dispersed across the U.S. innovation ecosystem and lack a national mission.62 A national GenAI-enabled S&T Discovery Platform moonshot could combine talent and resources across these efforts to build a proprietary model from scratch or license and/or fine-tune commercial or open-source LLMs. The U.S. government could house such an effort at one of DOE’s National Labs to provide access to computing resources and S&T expertise.
- National Medical Shield: The convergence of GenAI and biotechnology creates both immense opportunities and asymmetrically dangerous risks. To step comfortably into this world, the United States needs a robust, interagency system for identifying and rapidly responding to any potential biothreat. As called for in SCSP’s National Action Plan for U.S. Leadership in Biotechnology, the U.S. government should field a 24/7 national medical shield (“MedShield”) housed in the Department of Defense (DoD) that functions as a NORAD-like incident command system.63 GenAI not only increases the urgency of such an effort, but offers a practical tool to stitch together existing innovations in this space across the public-private spectrum in the United States and with allies and partners.
- Accelerate Other Moonshots with GenAI: GenAI should be leveraged as a tool of first resort to spur moonshots in strategic technology sectors and boost existing moonshot efforts. For example, GenAI could help streamline network communications to achieve pervasive, interoperable connectivity nationwide by 2035.64 GenAI-enabled design and process optimization can also help accelerate and integrate efforts to build a fully synthetic cell and move the field of biotechnology towards a paradigm of reliable and routine bioengineering.65 GenAI could also propel new moonshots for challenges and opportunities such as personalized education at scale, global fresh water availability, or fast-charging batteries for electric vehicles. Finally, GenAI could augment moonshot efforts underway such as President Biden’s Cancer Moonshot.66
Fueling the New Course to the Endless Frontier
Vannevar Bush, the American engineer, inventor, and science administrator, wrote in Science: the Endless Frontier about the crucial role of science in national development, underscoring the need for increased government support for scientific research in the United States.67 The current GenAI moment is an opportunity to get federal R&D spending – the lifeblood of innovation – back on track.68 Even though private industry should be credited in this most recent wave of GenAI innovations, we should not forget the role the U.S. government played in some of the foundational research that made GenAI what it is today. In the 1960s, partly driven by the Apollo Program and America’s mission to reach the Moon, the federal government funded the majority of total U.S. research and development, and for decades after, government R&D funding continued to play a role in the development of various transformative technologies.69 Today, private industry is the big R&D spender, while the share of federal R&D dollars has fallen drastically, diminishing the government’s ability to set strategic R&D priorities.70 GenAI’s revolutionary power could further tilt the geometry of innovation71 towards industry as the primary driver of R&D while academia and government risk falling behind.72 In pursuit of Bush’s “endless frontier,” Congress and the White House should adopt measures to rebalance America’s geometry of innovation and allocate new resources to bolster the nation’s innovation power so that the government can begin reasserting its role in creating future waves of innovation.
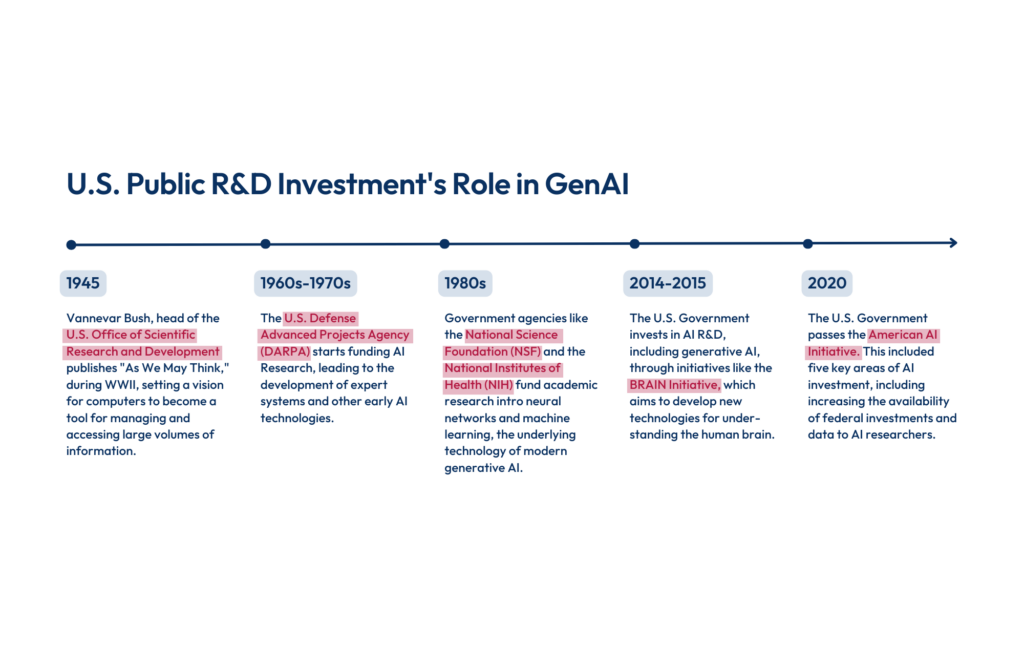
5. Get Back to the Basics
Academia is the primary performer of basic research, and industry often builds upon the discoveries made by national labs and universities to translate them into practical applications and new technologies.73 GenAI is changing every step of scientific discovery and its impact on basic research will be revolutionary across scientific fields and tech sectors.74 To harness GenAI’s convergence with other scientific areas, the government should increase funding for government basic research.
- For Fiscal Year 2024, Congress should fully appropriate the “Science” portion of the CHIPS and Science Act, as around $5 billion remains unfunded today.75 This would increase the budgets for critical federal R&D agencies: the National Science Foundation, the National Institute of Standards and Technology, and the Department of Energy’s Office of Science.76 These agencies could use an increase in funding to provide for the automatic translation of PRC research papers to direct projects toward areas where the United States falls behind the leading edge and for new directives aimed at creating high-quality datasets for every scientific field with which GenAI converges, which would enable AlphaFold-like accelerators of scientific discovery.77 With the emergence of GenAI, increasing federal funding for basic research will bring dividends to S&T innovation for generations to come.
6. Expand Overall Government Investments in R&D
The private sector is likely to remain – and should remain – at the cutting-edge of AI research and commercial applications. The U.S. government, however, should invest resources to ensure a wide set of actors can contribute to the field. These resources should include providing academic and non-profit researchers access to computing power, as well as creating a demand for exploratory research and development that is in the nation’s interest.78 As GenAI is poised to add trillions to the global economy, the U.S. government should direct more R&D funding towards priority strategic technology sectors, including AI broadly, to jumpstart GenAI’s catalytic potential to accelerate innovation itself.79
- The U.S. government should aim to increase federal R&D funding to one percent of the total U.S. GDP by 2026. Reaching this target means federal R&D funding would need to increase by approximately $83 billion over the next two years. To this end, Congress should, in the short term, fully appropriate the R&D spending target outlined in the President’s 2024 Budget Request.80 Subsequent budget requests starting with Fiscal Year 2025 should dramatically increase R&D spending, including raising non-defense AI R&D by $14.2 billion.81 Such a jolt to R&D spending would equip the federal government with the resources to reach its GenAI moonshots, spur investment from other sources across the innovation cycle,82 and help recalibrate the ecosystem for the GenAI moment.
7. Create a More Agile Grant Process
The federal funding process should become faster and more flexible so researchers can spend more time solving today’s pressing problems instead of applying and waiting for grant approvals.83
- Drawing inspiration from novel, agile funding models that have bubbled up across the innovation ecosystem, such as Fast Grants,84 the National Science Foundation (NSF) should develop a GenAI-enabled rapid grant program through which it should shepherd a portion of the existing funding NSF distributes across the federal R&D enterprise.85
8. Move Beyond an Episodic Competition Budget Cycle
As the GenAI flywheel takes off, Congress needs to be ready to put its foot on the gas when new innovation opportunities demand it.
- To further remove the bureaucratic red tape that hampers sustainable and long-term R&D, Congress could establish an annual technology innovation fund modeled after the DoD “Overseas Contingency Operations”86 or pursue sector-specific R&D funding models similar to aspects of the CHIPS and Science Act that would provide long-term investments and lead to a sustained committed strategic technology targets.87
Conclusion
America’s early lead in GenAI today proves the enduring strength of the innovation ecosystem Vannevar Bush helped build. Yet general purpose technologies are defined by their long-term diffusion, and technology leadership is determined by societies’ willingness to lean into their disruptive potential, adapt, and adopt. The generative character of GenAI — unique among the GPTs to date — means that the nations that first use this technology to out-innovate their rivals and fully exercise their innovation power are unlikely to be caught. This flywheel has started, but steering it towards national security and national competitiveness will require gathering the strengths across our innovation ecosystem for public-private strategy, coordination, and investment. The government may not be in the driver’s seat, but it must have a hand on the wheel. The White House and Congress should set the nation on a course to lead the AI era by organizing, goal-setting, and right-sizing R&D for AI-enabled innovation.
Endnotes
- Innovation power is a nation’s capacity to invent, adopt, and adapt to new technologies. See Eric Schmidt, Innovation Power: Why Technology Will Define the Future of Geopolitics, Foreign Affairs (2023); Dan Wang, China’s Hidden Tech Revolution: How Beijing Threatens U.S. Dominance, Foreign Affairs (2023).
- Francis Bacon, Novum Organum, Open Court at 63 (1994).
- Joel Mokyr, The Lever of Riches: Technological Creativity and Economic Progress, Oxford University Press at 145-72 (1992).
- Noah Shinn, et al., Reflexion: Language Agents with Verbal Reinforcement Learning, arXiv (2023).
- Nat Friedman, Introducing GitHub Copilot: Your AI Pair Programmer, Github Blog (2021); Yiwen Lu, What to Know About ChatGPT’s New Code Interpreter Feature, New York Times (2023).
- The innovation flywheel concept has been used to characterize periods of acceleration in digital innovation. See Charles Gildehaus, et al.,Powering the Innovation Flywheel in the Digital Era, Boston Consulting Group (2021).
- Sébastien Bubeck, et al., Sparks of Artificial General Intelligence: Early Experiments with GPT-4. arXiv (2023). After much debate over the definitions, the commissioners of the NSCAI explained something between today and AGI as “more general artificial intelligence.” This term captured the state of innovation between narrow AI and the theoretical concept of AGI. See Final Report, National Security Commission on Artificial Intelligence at 35-36 (2021).
- See e.g., Linda Geddes, DeepMind Uncovers Structure of 200m Proteins in Scientific Leap Forward, The Guardian (2022). For a definition of innovation see Fred Gault, Defining and Measuring Innovation in all Sectors of the Economy, Research Policy (2018).
- Marco Ianisiti & Karim R. Lakhani, Competing in the Age of AI: Strategy and Leadership When Algorithms and Networks Run the World, Harvard Business Press (2020).
- Madhumita Murgia, Why Computer-Made Data is Being Used to Train AI Models, Financial Times (2023).
- Michael Chui, et al., The Economic Potential of Generative AI: The Next Productivity Frontier, McKinsey & Company (2023); See also Vildana Hajric & Lu Wang, AI Proves Mightier Than the Fed for Stocks Divorced From Economy, Bloomberg (2023).
- Eric Schmidt, This is How AI Will Transform the Way Science Gets Done, MIT Technology Review (2023).
- AlphaFold Reveals the Structure of the Protein Universe, DeepMind (2022).
- Jamie Smyth, Biotech Begins Human Trials of Drug Designed by Artificial Intelligence, Financial Times (2023).
- Oana Stroe, Case study: AlphaFold Uses Open Data and AI to Discover the 3D Protein Universe, European Molecular Biology Laboratory (2023).
- Jason Dorrier, Here’s Why Google’s Deepmind’s Gemini Algorithm Could Be Next-Level AI, Singularity Hub (2023); NVIDIA Announces Digital Twin Platform for Scientific Computing, Digital Engineering (2022).
- How We Harness Industrial Generative AI for Optimization, Zapata AI (last accessed 2023).
- Tiechui Yao, et al., VenusAI: An Artificial Intelligence Platform for Scientific Discovery on Supercomputers, Journal of Systems Architecture (2022).
- As one early example of such capabilities, see Yiwen Lu, What to Know About ChatGPT’s New Code Interpreter Feature, New York Times (2023).
- Ron Miller, Generative AI Could Transform the Way We Interact with Enterprise Software, TechCrunch (2023).
- Pudi Dhilleswararao, et al., Efficient Hardware Architectures for Accelerating Deep Neural Networks: Survey, IEEE Access (2022); Adi Fuchs, AI Accelerators — Part IV: The Very Rich Landscape, Medium.com (2021).
- AI for Discovery and Self-Driving Lab, The Matter Lab (last accessed 2023); George Anadiotis, Red Hat and IBM Team Up to Enhance Alops with an Open Source Project, Venture Beat (2022).
- Chris Stokel-Walker & Richard Van Noorden, What ChatGPT and Generative AI Mean for Science, Nature (2023).
- Rob Toews, Synthetic Data is About to Transform Artificial Intelligence, Forbes (2022).
- Chris Stokel-Walker & Richard Van Noorden, What ChatGPT and Generative AI Mean for Science, Nature (2023).
- Reed Albergotti, Tome, an AI-Powered Storytelling Tool, Catches Fire with Gen Z, Semafor (2023).
- Ishwar Parulkar, How Generative AI will Transform the Telco Industry—And Where It Won’t, Light Reading (2023).
- Tim Keary, Oasis Labs and Equifax Turn to Blockchain to Verify Web3 User Identities, VentureBeat (2022).
- Introducing Microsoft Security Copilot: Empowering Defenders at the Speed of AI, Microsoft (2023).
- Matteo Manica, et al., Accelerating Material Design with the Generative Toolkit for Scientific Discovery, Nature (2023).
- Hector G. Martin, et al., Perspectives for Self-Driving Labs in Synthetic Biology, Current Opinion in Biotechnology (2023); Eric Schmidt, This is How AI Will Transform the Way Science Gets Done, MIT Technology Review (2023).
- John Jumper & Demis Hassabis, Protein Structure Predictions to Atomic Accuracy with AlphaFold, Nature Methods (2022); Abel Chandra, et al., Transformer-Based Deep Learning for Predicting Protein Properties in the Life Sciences, Computational and Systems Biology (2023); Jiaying Huang, et al., Discovery of Deaminase Functions by Structure-Based Protein Clustering, Cell (2023).
- Gary Liu, et al., Deep Learning-Guided Discovery of an Antibiotic Targeting Acinetobacter Baumannii, Nature Chemical Biology (2023); Vanessa Braunstein, Build Generative AI Pipelines for Drug Discovery with NVIDIA BioNeMo Service, NVIDIA Developer (2023).
- Generating ‘Smarter’ Biotechnology, Nature Biotechnology (2023).
- Spencer Acain, Reduce the Carbon Footprint of Manufacturing with Artificial Intelligence, Siemens (2022).
- Amit Katwala, DeepMind has Trained an AI to Control Nuclear Fusion, Wired (2022); Jonas Degrave, et al., Magnetic Control of Tokamak Plasmas Through Deep Reinforcement Learning, Nature (2022).
- Ginger Gardiner, Brighton Science Unveils BConnect: The World’s First Surface Intelligence Platform, CompositesWorld (2023).
- Mark Bergen, DeepMind Alum Wants to Use AI to Speed the Development of Green Materials, BNN Bloomberg (2023).
- Kevin Roose, Aided by AI Language Models, Google’s Robots Are Getting Smart, New York Times (2023).
- For examples from the National Security Council to the War Industries Board, see Hal Brands, The Twilight Struggle, Yale University Press at 174-78 (2022); Mobilizing America’s Economy and Society, U.S. World War I Centennial Commission (last accessed 2022).
- SCSP has previously outlined the shortcomings of current organizations’ capacity to serve as this institutional home. See Harnessing the New Geometry of Innovation, Special Competitive Studies Project at 52 (2022).
- Final Report, National Security Commission on Artificial Intelligence at 166 (2021); Harnessing the New Geometry of Innovation, Special Competitive Studies Project at 51 (2022).
- In 2022, Congress considered legislation for both a Technology Competitiveness Council (TCC) and Office of Global Competition Analysis (OCA). H.R. 8027, To Establish within the Executive Office of the President a Technology Competitiveness Council (2022); Courtney Albon, Lawmakers Propose ‘Technology Competitiveness Council’ to Champion US Innovation, C4ISRNet (2022); S. 4368, American Technology Leadership Act of 2022 (2022); Daniel Flatley, Senators Wary of China’s Tech Prowess Seek Competition Office, Bloomberg (2022). More recently, in 2023, the U.S. Senate has also introduced a legislative bill to establish an OCA. S.1873, Global Technology Leadership Act (2023).
- Harnessing the New Geometry of Innovation, Special Competitive Studies Project at 49 (2022).
- Ryan Heath, Schumer, Humbled by AI, Crafts Crash Course for Senate, Axios (2023).
- DOD Announces Establishment of Generative AI Task Force, U.S. Department of Defense (2023).
- The President’s Council of Advisors on Science & Technology (PCAST) and federal advisory committees such as the Department of Commerce’s National Artificial Intelligence Advisory Committee (NAIAC) are useful organizational models, but are limited in their reach and/or scope across the interagency and government department leaders.
- Final Report, National Security Commission on Artificial Intelligence at 127 (2021).
- The national laboratory system consists of 17 labs located across the United States that fall under the Department of Energy’s directive. Each lab performs leading-edge research in pursuit of the Department’s and the nation’s science and national security priorities, and maintains “unique scientific user facilities” and supercomputing capabilities. See America’s National Laboratory System, U.S. Department of Energy (2017).
- To expand public-private partnerships, the DOE would most likely have to take funding “out-of-hide,” creating a need to also increase funding for the department overall. “Out-of-hide” refers to funding a program out of existing, authorized and appropriated department funds. See DAU Glossary, Defense Acquisition University, (last accessed 2023).
- Future “front door/back door” public private partnerships could look like Lawrence Livermore National Laboratory’s AI Innovation Incubator that serves as a collaboration hub to connect the lab’s research and development with external academic and industry stakeholders like IBM. See AI Innovation Incubator, Lawrence Livermore National Laboratory (last accessed 2023).
- Billy Perrigo, AI Is as Risky as Pandemics and Nuclear War, Top CEOs Say, TIME (2023)
- Iansiti and Lakhani describe the “AI Factory” as the scalable decision engine that powers the digital operating model of the twenty-first century firm, enabled by AI-powered data gathering, analytics, and decision-making. See Marco Iansiti & Karim R. Lakhani, Competing in the Age of AI: Strategy and Leadership When Algorithms and Networks Run the World, Harvard Business Press at 53-54 (2020).
- Will Knight, Google DeepMind CEO Demis Hassabis Says Its Next Algorithm Will Eclipse ChatGPT, Wired (2023).
- Stephan Zheng, et al., The AI Economist: Taxation Policy Design via Two-Level Deep Multiagent Reinforcement Learning, Science Advances (2022).
- Stanley McChrystal & Anshu Roy, AI Has Entered the Situation Room, Foreign Policy (2023).
- David Silver, et al., Mastering the Game of Go Without Human Knowledge, Nature (2017).
- Such a model could stitch together models used by relevant departments and agencies for economic, military, diplomatic, information, and technical domains, and be fused with real-time open-source intelligence.
- A national program would need to include a full-time, competent national mission manager capable of integrating various private sector capabilities and facilitating interagency coordination; a legal framework for IP licensing across numerous private companies; a transition plan to departments/agencies who are not (all) looking for this capability; and data sharing, security, storage, and transport for a whole of government platform.
- Generative AI: Understanding Generative AI and How it Will Fundamentally Transform Our World, Accenture (last accessed 2023).
- For example, the technique of cognitive simulation (CogSim) is being used by researchers at Lawrence Livermore National Laboratory “to find large-scale structures in big data sets, teach existing models to better mirror experimental results, and create a feedback loop between experiments and models that accelerates research advances.” Cognitive Simulation Supercharges Scientific Research, Lawrence Livermore National Laboratory (2022).
- Jonathan Carter, et al., Advanced Research Directions on AI for Science, Energy, and Security, U.S. Department of Energy (2023).
- Innovation categories that a MedShield would combine include—but are not limited to—rapid vaccines, rapid therapeutics, a global biothreat “radar,” advanced AI-enabled modeling to facilitate product development and testing, rapid manufacturing technologies, and an enhanced trial system that speeds up effectiveness and efficiency in the delivery of medical solutions to citizens. National Action Plan for U.S. Leadership in Biotechnology, Special Competitive Studies Project at 8 (2023).
- National Action Plan for U.S. Advantage in Advanced Networks, Special Competitive Studies Project at 6 (2023).
- National Action Plan for US Leadership in Biotechnology, Special Competitive Studies Project at 8-9 (2023).
- The White House, The President and First Lady’s Cancer Moonshot (last accessed 2023).
- Vannevar Bush, Science The Endless Frontier, National Science Foundation (1945).
- Current federal R&D funding falls below targets recommended by the National Security Commission on Artificial Intelligence. See Final Report, National Security Commission on Artificial Intelligence at 188 (2021); Supplement to the President’s FY 2023 Budget, National Science and Technology Council (2022); and Chapter 6: Research and Development, Analytical Perspectives, Office of Management and Budget (2023).
- Research and Development: U.S. Trends and International Comparisons, Science and Engineering Indicators, National Science Board (2022).
- Research and Development: U.S. Trends and International Comparisons, Science and Engineering Indicators, National Science Board (2022).
- The geometry of innovation refers to the addition of private capital and a web-enabled “crowd” to Vannevar Bush’s “Triangle of Innovation” which included industry, academia, and government. Bush’s triangle supported U.S. technology leadership throughout the Cold War, but it has evolved to include the new actors we see within the ecosystem today. See Harnessing the New Geometry of Innovation, Special Competitive Studies Project at 23 (2022).
- Research and Development: U.S. Trends and International Comparisons, Science and Engineering Indicators, National Science Board (2022).
- Research and Development: U.S. Trends and International Comparisons, Science and Engineering Indicators, National Science Board (2022); Rebecca Mandt, et al., Federal R&D Funding: The Bedrock of National Innovation, MIT Science Policy Review (2020); The Future Postponed: Why Declining Investment in Basic Research Threatens a U.S. Innovation Deficit, MIT Committee to Evaluate the Innovation Deficit (2015).
- Generative AI can help researchers derive answers from vast amounts of data, information, and existing knowledge quickly, breaking through bottlenecks within the scientific process and leading to more “eureka moments.” Engaging with a broad range of information is necessary for discovery and innovation. See John R. Smith & Matteo Manica, How Generative AI Models Can Fuel Scientific Discovery, IBM (2022); Michael Park, et al., Papers and Patents Are Becoming Less Disruptive Over Time, Nature (2023).
- CHIPS & Science Funding Update: FY 2023 Omnibus, FY 2024 Budget Both Short by Millions, Federation of American Scientists (2023).
- CHIPS & Science Funding Update: FY 2023 Omnibus, FY 2024 Budget Both Short by Millions, Federation of American Scientists (2023).
- Whereas the scientific community would benefit from translated PRC research papers for fundamental science, policymakers would also benefit from an increased awareness of the PRC’s technological progress. Translating research papers for policymakers could also be a function of the proposed Office of Global Competition Analysis (OCA). Additionally, for more information about datasets for scientific discovery see Eric Schmidt, This is How AI Will Transform the Way Science Gets Done, MIT Technology Review (2023).
- Some scientific projects are in the public interest and therefore may not receive funding from non-federal sources. Areas of scientific research that have intangible value, involve high levels of risk, and have uncertain outcomes sometimes offer little financial or academic incentives for researchers to pursue. See Eric Schmidt, This is How AI Will Transform the Way Science Gets Done, MIT Technology Review (2023); The Future Postponed: Why Declining Investment in Basic Research Threatens a U.S. Innovation Deficit, MIT Committee to Evaluate the Innovation Deficit (2015).
- For GenAI’s monetary impact on the global economy see Michael Chui, et al., The Economic Potential of Generative AI: The Next Productivity Frontier, McKinsey & Company (2023).
- Chapter 6: Research and Development, Analytical Perspectives, Office of Management and Budget (2023).
- This figure is in line with NSCAI’s previous recommendations. Final Report, National Security Commission on Artificial Intelligence at 188 (2021). See also Supplement to the President’s FY 2023 Budget, National Science and Technology Council, NITRD (2022).
- Raising federal R&D funding by $1 would increase total U.S. R&D expenditures by $8 by spurring private investment. Welcome to the Machine: A Comparative Assessment of the USA and China to 2035 Focusing on the Role of Technology in the Economy,Fathom Financial Consulting Limited (2022).
- M. Anthony Mills, Fix Science, Don’t Just Fund It, Innovation Frontier Project (2021).
- See Fast Funding for COVID-19 Science, Fast Grants (last accessed 2023); Derek Thompson, Silicon Valley’s New Obsession: Science Funding, The Atlantic (2022).
- The federal government is currently taking steps to streamline and simplify the federal funding process, like modernizing iEdison, the government’s reporting system for federal R&D funding. In the future, iEdison could be enabled by artificial intelligence which would continue to speed up the reporting system, help track progress toward national goals, and be a resource for agencies to help decide where to invest. See Fact Sheet: Amidst Manufacturing Boom, President Biden Will Sign an Executive Order on Federal Research and Development in Support of Domestic Manufacturing and United States Jobs to Encourage “Invent it Here, Make it Here” in Industries of the Future, The White House (2023).
- The Overseas Contingency Operations is a separate fund from the DoD’s base funding, allocating funding for additional support towards emergencies. FY2022 NDAA: Overseas Contingency Operations, Congressional Research Service (2022).
- This annual technology innovation fund would be along the lines of the CHIPS and Science Act, but less episodic. Funding could be steered by a public-private technology strategy group run by the NSC or a future TCC. This funding mechanism could help move some federal R&D funding away from one year budget cycles. See Eric Schmidt, Innovation Power: Why Technology Will Define the Future of Geopolitics, Foreign Affairs (2023).